Schneider Electric – Schneider Electric and Compass Datacenters Expand Partnership with $3 Billion Multi-Year Data Center Technology Agreement
- Agreement between the companies is an expansion of an existing partnership to manufacture prefabricated modular data center solutions
- The integration of supply chains between companies will deliver finished goods more quickly, with predictability, and at a lower cost
BOSTON – November 9, 2023 – Schneider Electric, the leader in the digital transformation of energy management and automation, today announced at its Capital Markets Day meeting with investors a $3 billion multi-year agreement with Compass Datacenters. The agreement extends the companies’ existing relationship that integrates their respective supply chains to manufacture and deliver prefabricated modular data center solutions.
The skyrocketing generation and consumption of data, in large part due to the burgeoning AI market, requires a new breed of data centers that can be standardized in their design, manufactured efficiently, and delivered more quickly at a lower cost.
Since the inception of the initial agreement between the companies, Schneider Electric has manufactured and delivered about 150 modular data center solutions to Compass from its West Chester, OH operation. As demand for more data center solutions increase, the 110,000 square-foot Red Oak, TX operation—which the companies jointly announced in the summer—will also be used to support projected growth.
“We are extremely grateful for the unwavering support and unparalleled expertise that Schneider Electric has already provided in supplying us with pre-fabricated data center power rooms,” said Chris Crosby, CEO, Compass Datacenters. “Our collaborative efforts will allow us to meet the increasing demands from our customers to deliver cutting-edge data center solutions in an innovative manner.”
“We are proud that Compass chose Schneider Electric as their data center partner because we can provide the infrastructure they require in a predictable and reliable manner,” said Aamir Paul, President, Schneider Electric North America. “The extension of our agreement to continue innovating will address future demands to serve customers in industries ranging from cloud and service providers to semiconductors and EV’s, healthcare and telecommunication, among others.”
Through this unique 5-year vendor-supplier agreement, Schneider Electric and Compass Datacenters can deliver on the promise of scalable, modular data centers that offer a simplified design, streamlined manufacturing, and the ability to be deployed easily across many environments.
SourceSchneider Electric
EMR Analysis
More information on Schneider Electric: See the full profile on EMR Executive Services
More information on Peter Herweck (Chief Executive Officer, Schneider Electric): See the full profile on EMR Executive Services
More information on Annette Clayton (Chief Executive Officer and President, North America Operations, Schneider Electric): See the full profile on EMR Executive Services
More information on Aamir Paul (Member of the Executive Committee and Executive Vice President North America Operations, Schneider Electric): See the full profile on EMR Executive Services
More information on Compass Datacenters: http://www.compassdatacenters.com/ + Compass Datacenters, one of Inc. Magazine’s 5000 fastest growing companies, designs and constructs data centers for some of the world’s largest hyperscalers and cloud providers on campuses across the globe. Our corporate culture is predicated on continual improvement and innovation and has enabled us to marry technology with modern manufacturing methods to enhance our ability to consistently deliver our customer’s projects faster, with no sacrifices in quality. Since our inception, our sustainability efforts have encompassed the entire data center from its design to its post-delivery performance, including the efficient use of land, water-free cooling and a focus on Green House Gas reduction in the materials used to build our facilities and in their operation. Compass embraces a long-term perspective with the financial strength and operating expertise of investors Brookfield Infrastructure and Ontario Teachers’ Pension Plan.
More information on Chris Crosby (Chief Executive Officer, Compass Datacenters): https://www.compassdatacenters.com/about/leadership/ + https://www.linkedin.com/in/chris-crosby-3542b71/
EMR Additional Notes:
- AI – Artificial Intelligence:
- https://searchenterpriseai.techtarget.com/definition/AI-Artificial-Intelligence +
- Artificial intelligence is the simulation of human intelligence processes by machines, especially computer systems. Specific applications of AI include expert systems, natural language processing, speech recognition and machine vision.
- As the hype around AI has accelerated, vendors have been scrambling to promote how their products and services use AI. Often what they refer to as AI is simply one component of AI, such as machine learning. AI requires a foundation of specialized hardware and software for writing and training machine learning algorithms. No one programming language is synonymous with AI, but a few, including Python, R and Java, are popular.
- In general, AI systems work by ingesting large amounts of labeled training data, analyzing the data for correlations and patterns, and using these patterns to make predictions about future states. In this way, a chatbot that is fed examples of text chats can learn to produce lifelike exchanges with people, or an image recognition tool can learn to identify and describe objects in images by reviewing millions of examples.
- AI programming focuses on three cognitive skills: learning, reasoning and self-correction.
- What are the 4 types of artificial intelligence?
- Type 1: Reactive machines. These AI systems have no memory and are task specific. An example is Deep Blue, the IBM chess program that beat Garry Kasparov in the 1990s. Deep Blue can identify pieces on the chessboard and make predictions, but because it has no memory, it cannot use past experiences to inform future ones.
- Type 2: Limited memory. These AI systems have memory, so they can use past experiences to inform future decisions. Some of the decision-making functions in self-driving cars are designed this way.
- Type 3: Theory of mind. Theory of mind is a psychology term. When applied to AI, it means that the system would have the social intelligence to understand emotions. This type of AI will be able to infer human intentions and predict behavior, a necessary skill for AI systems to become integral members of human teams.
- Type 4: Self-awareness. In this category, AI systems have a sense of self, which gives them consciousness. Machines with self-awareness understand their own current state. This type of AI does not yet exist.
- Machine Learning:
- Developed to mimic human intelligence. It lets the machines learn independently by ingesting vast amounts of data and detecting patterns. Many ML algorithms use statistics formulas and big data to function.
- Type of artificial intelligence (AI) that allows software applications to become more accurate at predicting outcomes without being explicitly programmed to do so. Machine learning algorithms use historical data as input to predict new output values.
- Recommendation engines are a common use case for machine learning. Other popular uses include fraud detection, spam filtering, malware threat detection, business process automation (BPA) and Predictive maintenance.
- Classical machine learning is often categorized by how an algorithm learns to become more accurate in its predictions. There are four basic approaches: supervised learning, unsupervised learning, semi-supervised learning and reinforcement learning. The type of algorithm data scientists choose to use depends on what type of data they want to predict.
- Deep Learning:
- Subset of machine learning. Deep learning enabled much smarter results than were originally possible with machine learning. Consider the face recognition example.
- Deep learning makes use of layers of information processing, each gradually learning more and more complex representations of data. The early layers may learn about colors, the next ones learn about shapes, the following about combinations of those shapes, and finally actual objects. Deep learning demonstrated a breakthrough in object recognition.
- Deep learning is currently the most sophisticated AI architecture we have developed.
- Computer Vision:
- Computer vision is a field of artificial intelligence (AI) that enables computers and systems to derive meaningful information from digital images, videos and other visual inputs — and take actions or make recommendations based on that information.
- The most well-known case of this today is Google’s Translate, which can take an image of anything — from menus to signboards — and convert it into text that the program then translates into the user’s native language.
- Generative AI:
- Generative AI technology generates outputs based on some kind of input – often a prompt supplied by a person. Some GenAI tools work in one medium, such as turning text inputs into text outputs, for example. With the public release of ChatGPT in late November 2022, the world at large was introduced to an AI app capable of creating text that sounded more authentic and less artificial than any previous generation of computer-crafted text.
- https://searchenterpriseai.techtarget.com/definition/AI-Artificial-Intelligence +
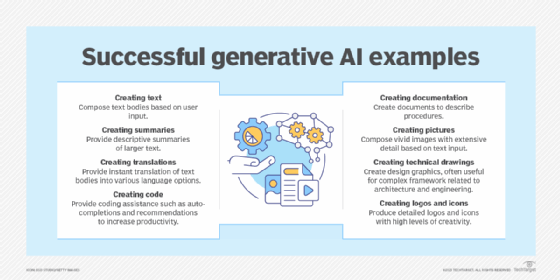
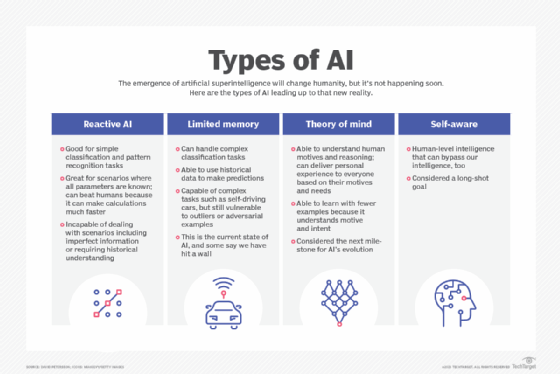
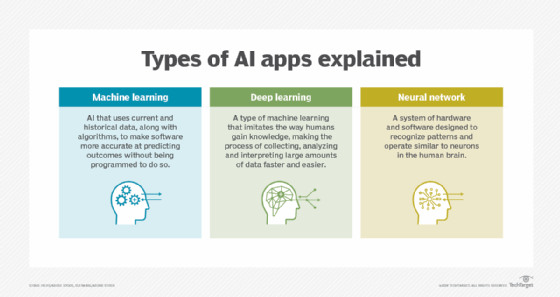
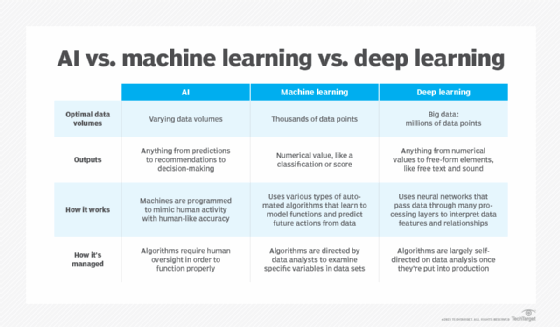
- Cloud Computing:
- Cloud computing is a general term for anything that involves delivering hosted services over the internet. … Cloud computing is a technology that uses the internet for storing and managing data on remote servers and then access data via the internet.
- Cloud computing is the on-demand availability of computer system resources, especially data storage and computing power, without direct active management by the user. Large clouds often have functions distributed over multiple locations, each location being a data center.
- Edge Computing:
- Edge computing is a form of computing that is done on site or near a particular data source, minimizing the need for data to be processed in a remote data center.
- Edge computing can enable more effective city traffic management. Examples of this include optimising bus frequency given fluctuations in demand, managing the opening and closing of extra lanes, and, in future, managing autonomous car flows.
- An edge device is any piece of hardware that controls data flow at the boundary between two networks. Edge devices fulfill a variety of roles, depending on what type of device they are, but they essentially serve as network entry — or exit — points.
- There are five main types of edge computing devices: IoT sensors, smart cameras, uCPE equipment, servers and processors. IoT sensors, smart cameras and uCPE equipment will reside on the customer premises, whereas servers and processors will reside in an edge computing data centre.
- In service-based industries such as the finance and e-commerce sector, edge computing devices also have roles to play. In this case, a smart phone, laptop, or tablet becomes the edge computing device.
- Edge Devices:
- Edge devices encompass a broad range of device types, including sensors, actuators and other endpoints, as well as IoT gateways. Within a local area network (LAN), switches in the access layer — that is, those connecting end-user devices to the aggregation layer — are sometimes called edge switches.
- Data Centers:
- A data center is a facility that centralizes an organization’s shared IT operations and equipment for the purposes of storing, processing, and disseminating data and applications. Because they house an organization’s most critical and proprietary assets, data centers are vital to the continuity of daily operations.
- Hyperscale Data Centers:
- The clue is in the name: hyperscale data centers are massive facilities built by companies with vast data processing and storage needs. These firms may derive their income directly from the applications or websites the equipment supports, or sell technology management services to third parties.