Avnet – Avnet and Syntiant Forge Strategic Alliance as Distribution Partners for Edge AI Technology
PHOENIX – Avnet (Nasdaq: AVT), a leading global technology distributor, has entered into a distribution agreement with Syntiant, a leader in edge AI deployment.
The partnership aims to combine Syntiant’s innovative hardware edge AI solutions with Avnet’s extensive distribution capabilities, potentially accelerating the adoption of AI technologies across various sectors.
Syntiant’s Neural Decision Processors bring ultra-low-power advanced sensing and decision-making to edge AI applications across a range of industries, including e-mobility, security, health, and industrial IoT, which aligns with Avnet’s diverse market reach. Independently verified to be 100x more power efficient and offer 10x the throughput when compared to existing low-power MCUs, Syntiant’s processors enable devices to detect motion, light, and vibration, recognize sounds, categorize images, identify objects, people, and animals, and engage in on-device AI-driven conversations. This broadens the scope of intelligent, responsive technologies in everyday electronic devices.
“Through our distribution partnership with Syntiant and the roll-out of our RASynBoard, Avnet brings accelerated edge AI, battery-powered remote sensor systems, industrial smart sensors, motor predictive maintenance, and always-on speech recognition capabilities to design engineers,” said Jim Beneke, vice president of Advanced Applications Group, Avnet. “This edge AI/ML solution underscores our commitment to quality, enabling widespread adoption of Syntiant’s advanced AI technologies and driving innovation across market sectors.
“Syntiant has a significant market presence with more than 50 million devices deployed using our technology,” said Syntiant CEO Kurt Busch. “Our partnership with Avnet will facilitate even more market expansion by leveraging Avnet’s distribution network to enhance customer access to Syntiant’s edge AI hardware technology, which is powering larger networks, while consuming significantly less power.”
More information on the RASynBoard can be found here. Syntiant will also have the board on display at CES 2024 in Las Vegas. To schedule a demo at CES contact info@syntiant.com.
SourceAvnet
EMR Analysis
More information on Avnet: See the full profile on EMR Executive Services
More information on Phil Gallagher (Chief Executive Officer, Avnet): See the full profile on EMR Executive Services
More information on Multicomp Pro by Avnet: See the full profile on EMR Executive Services
More information on Farnell (Farnell, Newark, element14): See the full profile on EMR Executive Services
More information on Chris Breslin (President, Farnell, Avnet): See the full profile on EMR Executive Services
More information on RASynBoard by Avnet: https://www.avnet.com/wps/portal/us/products/avnet-boards/avnet-board-families/rasynboard/ + Low power sensor-fusion wireless AI/ML module.
RASynBoard is a low-cost evaluation kit that includes the RASynBoard core module with an I/O board for prototyping and development.
RASynBoard is a tiny (25mm x 30mm), ultra-low power, edge AI/ML board, based on a Syntiant NDP120 Neural Decision Processor, a Renesas RA6M4 host MCU plus a power-efficient DA16600 Wi-Fi/BT combo module. The NDP120 subsystem with onboard digital microphone, IMU motion sensor and SPI Flash memory, achieves highly efficient processing of acoustic- and motion events. Battery and USB-C device connectors facilitate stand-alone use, while a compact under-board connector enables integration with custom OEM boards and additional sensors.
More information on Jim Beneke (Vice President, Advanced Applications Group, Avnet): See the full profile on EMR Executive Services
More information on Syntiant: https://www.syntiant.com/ + FFounded in 2017 and headquartered in Irvine, Calif., Syntiant Corp. is a leader in delivering hardware and software solutions for edge AI deployment. The company’s purpose-built silicon and hardware-agnostic models are being deployed globally to power edge AI speech, audio, sensor and vision applications across a wide range of consumer and industrial use cases, from earbuds to automobiles. Syntiant’s advanced chip solutions merge deep learning with semiconductor design to produce ultra-low-power, high performance, deep neural network processors. Syntiant also provides compute-efficient software solutions with proprietary model architectures that enable world-leading inference speed and minimized memory footprint across a broad range of processors. The company is backed by several of the world’s leading strategic and financial investors including Intel Capital, Microsoft’s M12, Applied Ventures, Robert Bosch Venture Capital, the Amazon Alexa Fund and Atlantic Bridge Capital.
More information on Kurt Busch (Chief Executive Officer, Syntiant): https://www.syntiant.com/leadership + https://www.linkedin.com/in/kfbusch/
More information on CES 2024 (Jan. 9-12, in Las Vegas – United States): https://www.ces.tech/about-ces.aspx + CES® is the most powerful tech event in the world — the proving ground for breakthrough technologies and global innovators. This is where brands get business done, meet new partners and where the industry’s sharpest minds take the stage to unveil their latest releases and boldest breakthroughs. Owned and produced by the Consumer Technology Association (CTA)®, CES is the only trade show that showcases the entire tech landscape at one event.
EMR Additional Notes:
- AI – Artificial Intelligence:
- https://searchenterpriseai.techtarget.com/definition/AI-Artificial-Intelligence +
- Artificial intelligence is the simulation of human intelligence processes by machines, especially computer systems. Specific applications of AI include expert systems, natural language processing, speech recognition and machine vision.
- As the hype around AI has accelerated, vendors have been scrambling to promote how their products and services use AI. Often what they refer to as AI is simply one component of AI, such as machine learning. AI requires a foundation of specialized hardware and software for writing and training machine learning algorithms. No one programming language is synonymous with AI, but a few, including Python, R and Java, are popular.
- In general, AI systems work by ingesting large amounts of labeled training data, analyzing the data for correlations and patterns, and using these patterns to make predictions about future states. In this way, a chatbot that is fed examples of text chats can learn to produce lifelike exchanges with people, or an image recognition tool can learn to identify and describe objects in images by reviewing millions of examples.
- AI programming focuses on three cognitive skills: learning, reasoning and self-correction.
- What are the 4 types of artificial intelligence?
- Type 1: Reactive machines. These AI systems have no memory and are task specific. An example is Deep Blue, the IBM chess program that beat Garry Kasparov in the 1990s. Deep Blue can identify pieces on the chessboard and make predictions, but because it has no memory, it cannot use past experiences to inform future ones.
- Type 2: Limited memory. These AI systems have memory, so they can use past experiences to inform future decisions. Some of the decision-making functions in self-driving cars are designed this way.
- Type 3: Theory of mind. Theory of mind is a psychology term. When applied to AI, it means that the system would have the social intelligence to understand emotions. This type of AI will be able to infer human intentions and predict behavior, a necessary skill for AI systems to become integral members of human teams.
- Type 4: Self-awareness. In this category, AI systems have a sense of self, which gives them consciousness. Machines with self-awareness understand their own current state. This type of AI does not yet exist.
- Machine Learning:
- Developed to mimic human intelligence. It lets the machines learn independently by ingesting vast amounts of data and detecting patterns. Many ML algorithms use statistics formulas and big data to function.
- Type of artificial intelligence (AI) that allows software applications to become more accurate at predicting outcomes without being explicitly programmed to do so. Machine learning algorithms use historical data as input to predict new output values.
- Recommendation engines are a common use case for machine learning. Other popular uses include fraud detection, spam filtering, malware threat detection, business process automation (BPA) and Predictive maintenance.
- Classical machine learning is often categorized by how an algorithm learns to become more accurate in its predictions. There are four basic approaches: supervised learning, unsupervised learning, semi-supervised learning and reinforcement learning. The type of algorithm data scientists choose to use depends on what type of data they want to predict.
- Deep Learning:
- Subset of machine learning. Deep learning enabled much smarter results than were originally possible with machine learning. Consider the face recognition example.
- Deep learning makes use of layers of information processing, each gradually learning more and more complex representations of data. The early layers may learn about colors, the next ones learn about shapes, the following about combinations of those shapes, and finally actual objects. Deep learning demonstrated a breakthrough in object recognition.
- Deep learning is currently the most sophisticated AI architecture we have developed.
- Computer Vision:
- Computer vision is a field of artificial intelligence (AI) that enables computers and systems to derive meaningful information from digital images, videos and other visual inputs — and take actions or make recommendations based on that information.
- The most well-known case of this today is Google’s Translate, which can take an image of anything — from menus to signboards — and convert it into text that the program then translates into the user’s native language.
- Generative AI:
- Generative AI technology generates outputs based on some kind of input – often a prompt supplied by a person. Some GenAI tools work in one medium, such as turning text inputs into text outputs, for example. With the public release of ChatGPT in late November 2022, the world at large was introduced to an AI app capable of creating text that sounded more authentic and less artificial than any previous generation of computer-crafted text.
- https://searchenterpriseai.techtarget.com/definition/AI-Artificial-Intelligence +
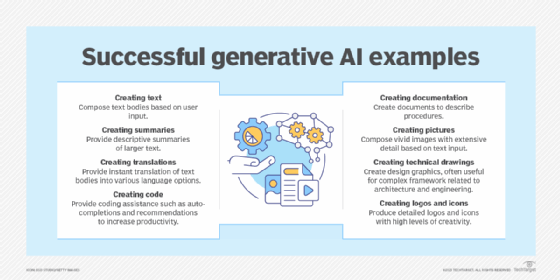
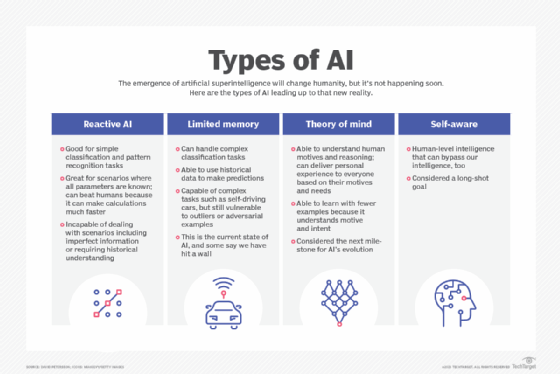
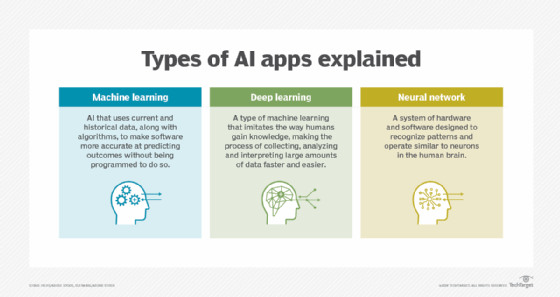
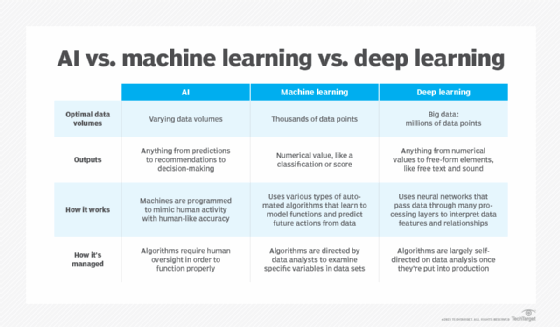
- Edge AI Technology:
- Edge artificial intelligence refers to the deployment of AI algorithms and AI models directly on local edge devices such as sensors or Internet of Things (IoT) devices, which enables real-time data processing and analysis without constant reliance on cloud infrastructure.
- Simply stated, edge AI, or “AI on the edge“, refers to the combination of edge computing and artificial intelligence to execute machine learning tasks directly on interconnected edge devices. Edge computing allows for data to be stored close to the device location, and AI algorithms enable the data to be processed right on the network edge, with or without an internet connection. This facilitates the processing of data within milliseconds, providing real-time feedback.
- Self-driving cars, wearable devices, security cameras, and smart home appliances are among the technologies that leverage edge AI capabilities to promptly deliver users with real-time information when it is most essential.
- Cloud Computing:
- Cloud computing is a general term for anything that involves delivering hosted services over the internet. … Cloud computing is a technology that uses the internet for storing and managing data on remote servers and then access data via the internet.
- Cloud computing is the on-demand availability of computer system resources, especially data storage and computing power, without direct active management by the user. Large clouds often have functions distributed over multiple locations, each location being a data center.
- Edge Computing:
- Edge computing is a form of computing that is done on site or near a particular data source, minimizing the need for data to be processed in a remote data center.
- Edge computing can enable more effective city traffic management. Examples of this include optimising bus frequency given fluctuations in demand, managing the opening and closing of extra lanes, and, in future, managing autonomous car flows.
- An edge device is any piece of hardware that controls data flow at the boundary between two networks. Edge devices fulfill a variety of roles, depending on what type of device they are, but they essentially serve as network entry — or exit — points.
- There are five main types of edge computing devices: IoT sensors, smart cameras, uCPE equipment, servers and processors. IoT sensors, smart cameras and uCPE equipment will reside on the customer premises, whereas servers and processors will reside in an edge computing data centre.
- In service-based industries such as the finance and e-commerce sector, edge computing devices also have roles to play. In this case, a smart phone, laptop, or tablet becomes the edge computing device.
- Edge Devices:
- Edge devices encompass a broad range of device types, including sensors, actuators and other endpoints, as well as IoT gateways. Within a local area network (LAN), switches in the access layer — that is, those connecting end-user devices to the aggregation layer — are sometimes called edge switches.
- Data Centers:
- A data center is a facility that centralizes an organization’s shared IT operations and equipment for the purposes of storing, processing, and disseminating data and applications. Because they house an organization’s most critical and proprietary assets, data centers are vital to the continuity of daily operations.
- Hyperscale Data Centers:
- The clue is in the name: hyperscale data centers are massive facilities built by companies with vast data processing and storage needs. These firms may derive their income directly from the applications or websites the equipment supports, or sell technology management services to third parties.
- IoT (Internet of Things):
- The Internet of Things (IoT) refers to a system of interrelated, internet-connected objects that are able to collect and transfer data over a wireless network without human intervention.
- Describes the network of physical objects—“things”—that are embedded with sensors, software, and other technologies for the purpose of connecting and exchanging data with other devices and systems over the internet.
- The Most Popular IoT Devices are:
- Smart watches are the most popular IoT devices. …
- Gaming consoles. …
- Smart TV sets and content streaming devices. …
- Voice control devices. …
- Printers. …
- Cameras. …
- Lighting appliances. …
- Smart thermostats.
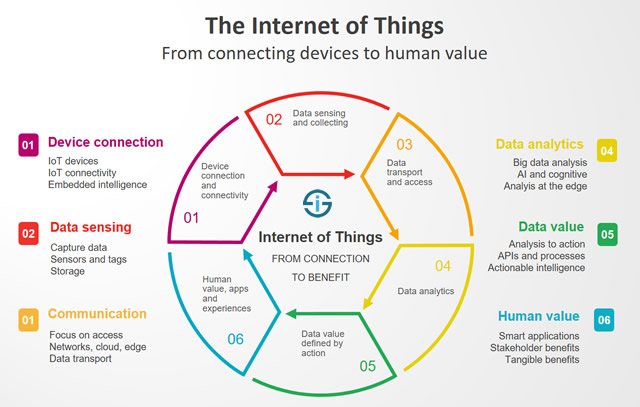
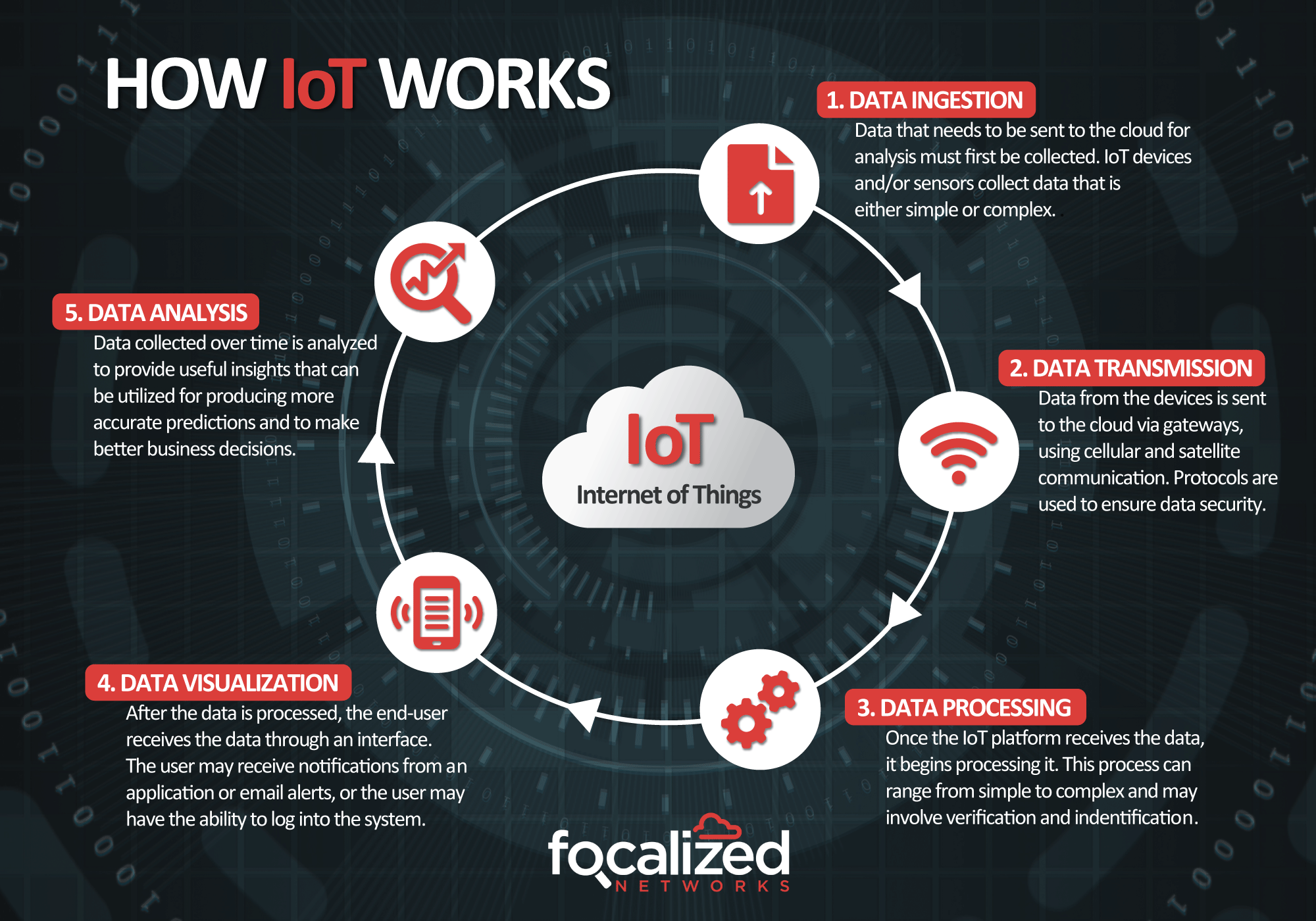
- IIoT (Industrial IoT):
- Industrial IoT (IIoT) involves collecting and analyzing sensor-generated data to support equipment monitoring and maintenance, production process analytics and control, and more. In manufacturing IT since 1989, ScienceSoft offers IIoT consulting and development to create secure IIoT solutions.
- xIoT (xTended Internet of Things):
- xIoT refers to the “xTended” Internet of Things. This xTended IoT category spans Enterprise IoT devices (cameras, printers, and door controllers), OT devices (like PLCs, HMI’s, and robotics) and Network devices (like switches, WiFi routers, and NAS).
- Low Power MCU (Micro controller):
- A microcontroller (MC, UC, or μC) or microcontroller unit (MCU) is a small computer on a single integrated circuit. A microcontroller contains one or more CPUs (processor cores) along with memory and programmable input/output peripherals.
- Analog Devices’ ultra low power (ULP) microcontroller allows edge nodes to intelligently process localized data with the smallest amount of system power needed. This allows customers to extend battery life and extend the time between charges allowing longer use.