Schneider Electric – Schneider Electric Collaborates with NVIDIA on Designs for AI Data Centers
- New reference designs will offer a robust framework for implementing NVIDIA’s accelerated computing platform within data centers
- Designs will optimize performance, scalability, and energy efficiency
Schneider Electric, the leader in the digital transformation of energy management and automation, today announced a collaboration with NVIDIA to optimize data center infrastructure and pave the way for groundbreaking advancements in edge artificial intelligence (AI) and digital twin technologies.
Schneider Electric will leverage its expertise in data center infrastructure and NVIDIA’s advanced AI technologies to introduce the first publicly available AI data center reference designs. These designs are set to redefine the benchmarks for AI deployment and operation within data center ecosystems, marking a significant milestone in the industry’s evolution.
With AI applications gaining traction across industries, while also demanding more resources than traditional computing, the need for processing power has surged exponentially. The rise of AI has spurred notable transformations and complexities in data center design and operation, with data center operators working to swiftly construct and operate energy-stable facilities that are both energy-efficient and scalable.
“We’re unlocking the future of AI for organizations,” said Pankaj Sharma, Executive Vice President, Secure Power Division & Data Center Business, Schneider Electric. “By combining our expertise in data center solutions with NVIDIA’s leadership in AI technologies, we’re helping organizations to overcome data center infrastructure limitations and unlock the full potential of AI. Our collaboration with NVIDIA paves the way for a more efficient, sustainable, and transformative future, powered by AI.”
Cutting-Edge Data Center Reference Designs
In the first phase of this collaboration, Schneider Electric will introduce cutting-edge data center reference designs tailored for NVIDIA accelerated computing clusters and built for data processing, engineering simulation, electronic design automation, computer-aided drug design, and generative AI. Special focus will be on enabling high-power distribution, liquid-cooling systems, and controls designed to ensure simple commissioning and reliable operations for the extreme-density cluster. Through the collaboration, Schneider Electric aims to provide data center owners and operators with the tools and resources necessary to seamlessly integrate new and evolving AI solutions into their infrastructure, enhancing deployment efficiency, and ensuring reliable life-cycle operation.
Addressing the evolving demands of AI workloads, the reference designs will offer a robust framework for implementing NVIDIA’s accelerated computing platform within data centers, while optimizing performance, scalability, and overall sustainability. Partners, engineers, and data center leaders can utilize these reference designs for existing data center rooms that must support new deployments of high-density AI servers and new data center builds that are fully optimized for a liquid-cooled AI cluster.
“Through our collaboration with Schneider Electric, we’re providing AI data center reference designs using next-generation NVIDIA accelerated computing technologies,” said Ian Buck, Vice President of Hyperscale and HPC at NVIDIA. “This provides organizations with the necessary infrastructure to tap into the potential of AI, driving innovation and digital transformation across industries.”
Future Roadmap
In addition to the data center reference designs,AVEVA, a subsidiary of Schneider Electric, will connect its digital twin platform to NVIDIA Omniverse, delivering a unified environment for virtual simulation and collaboration. This integration will enable seamless collaboration between designers, engineers, and stakeholders, accelerating the design and deployment of complex systems, while helping reduce time-to-market and costs.
“NVIDIA technologies enhance AVEVA’s capabilities in creating a realistic and immersive collaboration experience underpinned by the rich data and capabilities of the AVEVA intelligent digital twin,” said Caspar Herzberg, CEO of AVEVA. “Together, we are creating a fully simulated industrial virtual reality where you can simulate processes, model outcomes, and effect change in reality. This merging of digital intelligence and real-world outcomes has the potential to transform how industries can operate more safely, more efficiently and more sustainably.”
In collaboration with NVIDIA, Schneider Electric plans to explore new use cases and applications across industries and further its vision of driving positive change and shaping the future of technology.
More information will be available at Schneider Electric’s Innovation Summit Paris on April 3.
SourceSchneider Electric
EMR Analysis
More information on Schneider Electric: See the full profile on EMR Executive Services
More information on Peter Herweck (Chief Executive Officer, Schneider Electric): See the full profile on EMR Executive Services
More information on Pankaj Sharma (Executive Vice President, Secure Power Division & Data Center Business, Schneider Electric): See the full profile on EMR Executive Services
More information on AVEVA by Schneider Electric: https://www.aveva.com/en/ + AVEVA is a global leader in industrial software, sparking ingenuity to drive responsible use of the world’s resources. The company’s secure industrial cloud platform and applications enable businesses to harness the power of their information and improve collaboration with customers, suppliers and partners.
Over 20,000 enterprises in over 100 countries rely on AVEVA to help them deliver life’s essentials: safe and reliable energy, food, medicines, infrastructure and more. By connecting people with trusted information and AI-enriched insights, AVEVA enables teams to engineer efficiently and optimize operations, driving growth and sustainability.
Named as one of the world’s most innovative companies, AVEVA supports customers with open solutions and the expertise of more than 6,400 employees, 5,000 partners and 5,700 certified developers. With operations around the globe, AVEVA is headquartered in Cambridge, UK.
- 2022 Revenue: £1,185.3m at +44.5%
- 20,000+ customers
- in 100+ countries
- c. 6,500 employees
- 5,000+ partners
- 5,700 certified developers
More information on Caspar Herzberg (Member of the Executive Committee, Chief Executive Officer, AVEVA, Schneider Electric): See the full profile on EMR Executive Services
More information on the Schneider Electric Innovation Summit Paris 2024 (April 3-4, 2024 – Paris – France): https://www.se.com/ww/en/about-us/events/innovation-summit-world-tour.jsp + Discover what’s next in automation, electrification, and digitization. Come to get energized, network, and go hands-on with the technologies shaping the future of your business and industry.
More information on NVIDIA: https://www.nvidia.com/en-us/ + NVIDIA pioneered accelerated computing to tackle challenges no one else can solve. We engineer technology for the da Vincis and Einsteins of our time. Our work in AI is transforming 100 trillion dollars of industries—from gaming to healthcare to transportation—and profoundly impacting society.
Since its founding in 1993, NVIDIA (NASDAQ: NVDA) has been a pioneer in accelerated computing. The company’s invention of the GPU in 1999 sparked the growth of the PC gaming market, redefined computer graphics and ignited the era of modern AI. NVIDIA is now a full-stack computing company with data-center-scale offerings that are reshaping industry.
More information on Jensen Huang (Chief Executive Officer, NVIDIA): https://www.nvidia.com/en-us/about-nvidia/board-of-directors/jensen-huang/ + https://www.linkedin.com/in/jenhsunhuang/
More information on Ian Buck (Vice President, Hyperscale and HPC, NVIDIA): https://blogs.nvidia.com/blog/author/ian-buck/ + https://www.linkedin.com/in/ian-buck-19201315/
More information on Omniverse™ by NVIDIA: https://www.nvidia.com/en-us/omniverse/ + NVIDIA Omniverse™ is a platform of APIs, SDKs, and services that enable developers to easily integrate Universal Scene Description (OpenUSD) and RTX rendering technologies into existing software tools and simulation workflows for building AI systems.
EMR Additional Notes:
- AI – Artificial Intelligence:
- https://searchenterpriseai.techtarget.com/definition/AI-Artificial-Intelligence +
- Artificial intelligence is the simulation of human intelligence processes by machines, especially computer systems.
- As the hype around AI has accelerated, vendors have been scrambling to promote how their products and services use AI. Often what they refer to as AI is simply one component of AI, such as machine learning. AI requires a foundation of specialized hardware and software for writing and training machine learning algorithms. No one programming language is synonymous with AI, but well a few, including Python, R and Java, are popular.
- In general, AI systems work by ingesting large amounts of labeled training data, analyzing the data for correlations and patterns, and using these patterns to make predictions about future states. In this way, a chatbot that is fed examples of text chats can learn to produce lifelike exchanges with people, or an image recognition tool can learn to identify and describe objects in images by reviewing millions of examples.
- AI programming focuses on three cognitive skills: learning, reasoning and self-correction.
- What are the 4 types of artificial intelligence?
- Type 1: Reactive machines. These AI systems have no memory and are task specific. An example is Deep Blue, the IBM chess program that beat Garry Kasparov in the 1990s. Deep Blue can identify pieces on the chessboard and make predictions, but because it has no memory, it cannot use past experiences to inform future ones.
- Type 2: Limited memory. These AI systems have memory, so they can use past experiences to inform future decisions. Some of the decision-making functions in self-driving cars are designed this way.
- Type 3: Theory of mind. Theory of mind is a psychology term. When applied to AI, it means that the system would have the social intelligence to understand emotions. This type of AI will be able to infer human intentions and predict behavior, a necessary skill for AI systems to become integral members of human teams.
- Type 4: Self-awareness. In this category, AI systems have a sense of self, which gives them consciousness. Machines with self-awareness understand their own current state. This type of AI does not yet exist.
- Machine Learning (ML):
- Developed to mimic human intelligence, it lets the machines learn independently by ingesting vast amounts of data, statistics formulas and detecting patterns.
- ML allows software applications to become more accurate at predicting outcomes without being explicitly programmed to do so.
- ML algorithms use historical data as input to predict new output values.
- Recommendation engines are a common use case for ML. Other uses include fraud detection, spam filtering, business process automation (BPA) and predictive maintenance.
- Classical ML is often categorized by how an algorithm learns to become more accurate in its predictions. There are four basic approaches: supervised learning, unsupervised learning, semi-supervised learning and reinforcement learning.
- Deep Learning (DL):
- Subset of machine learning, Deep Learning enabled much smarter results than were originally possible with ML. Face recognition is a good example.
- DL makes use of layers of information processing, each gradually learning more and more complex representations of data. The early layers may learn about colors, the next ones about shapes, the following about combinations of those shapes, and finally actual objects. DL demonstrated a breakthrough in object recognition.
- DL is currently the most sophisticated AI architecture we have developed.
- Computer Vision (CV):
- Computer vision is a field of artificial intelligence that enables computers and systems to derive meaningful information from digital images, videos and other visual inputs — and take actions or make recommendations based on that information.
- The most well-known case of this today is Google’s Translate, which can take an image of anything — from menus to signboards — and convert it into text that the program then translates into the user’s native language.
- Machine Vision (MV):
- Machine Vision is the ability of a computer to see; it employs one or more video cameras, analog-to-digital conversion and digital signal processing. The resulting data goes to a computer or robot controller. Machine Vision is similar in complexity to Voice Recognition.
- MV uses the latest AI technologies to give industrial equipment the ability to see and analyze tasks in smart manufacturing, quality control, and worker safety.
- Computer Vision systems can gain valuable information from images, videos, and other visuals, whereas Machine Vision systems rely on the image captured by the system’s camera. Another difference is that Computer Vision systems are commonly used to extract and use as much data as possible about an object.
- Generative AI (GenAI):
- Generative AI technology generates outputs based on some kind of input – often a prompt supplied by a person. Some GenAI tools work in one medium, such as turning text inputs into text outputs, for example. With the public release of ChatGPT in late November 2022, the world at large was introduced to an AI app capable of creating text that sounded more authentic and less artificial than any previous generation of computer-crafted text.
- https://searchenterpriseai.techtarget.com/definition/AI-Artificial-Intelligence +
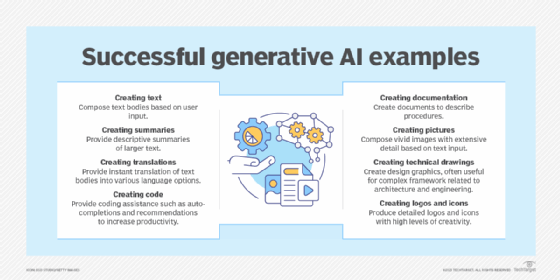
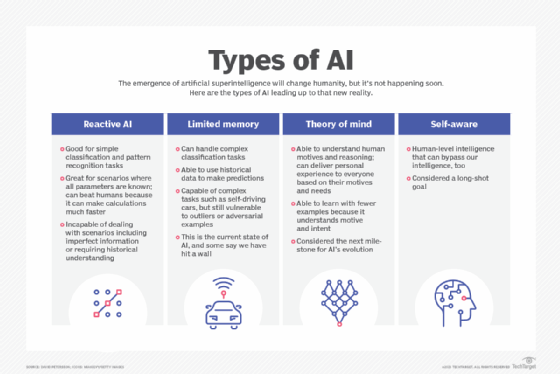
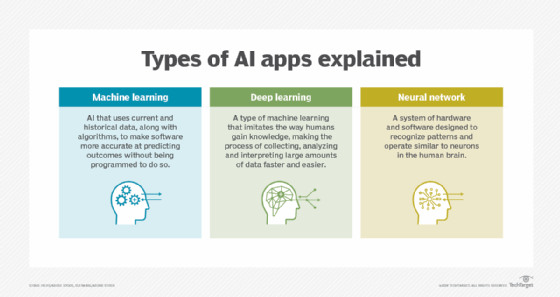
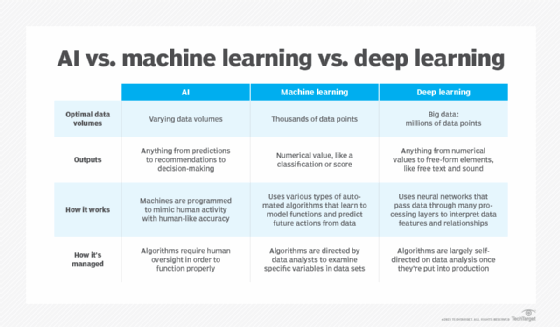
- Edge AI Technology:
- Edge artificial intelligence refers to the deployment of AI algorithms and AI models directly on local edge devices such as sensors or Internet of Things (IoT) devices, which enables real-time data processing and analysis without constant reliance on cloud infrastructure.
- Simply stated, edge AI, or “AI on the edge“, refers to the combination of edge computing and artificial intelligence to execute machine learning tasks directly on interconnected edge devices. Edge computing allows for data to be stored close to the device location, and AI algorithms enable the data to be processed right on the network edge, with or without an internet connection. This facilitates the processing of data within milliseconds, providing real-time feedback.
- Self-driving cars, wearable devices, security cameras, and smart home appliances are among the technologies that leverage edge AI capabilities to promptly deliver users with real-time information when it is most essential.
- Digital Twin:
- Digital Twin is most commonly defined as a software representation of a physical asset, system or process designed to detect, prevent, predict, and optimize through real time analytics to deliver business value.
- A digital twin is a virtual representation of an object or system that spans its lifecycle, is updated from real-time data, and uses simulation, machine learning and reasoning to help decision-making.