ABB – ABB invests in generative AI energy manager startup Edgecom
- ABB extends partnership with Edgecom, winner of its 2024 Startup Challenge
- Edgecom and ABB will collaborate on AI solutions for large industrial customers
ABB is investing in a strategic partnership with Edgecom Energy, the Toronto-based energy management startup. The company’s unique energy management platform uses artificial intelligence to help industrial and commercial users manage and reduce peaks in their power demand. It is the first in the market to use a generative AI copilot to optimize the user experience.
The partnership involves a minority investment in Edgecom through ABB Electrification Ventures, the venture capital arm of ABB Electrification. ABB Electrification Ventures is part of the group-wide ABB Ventures framework, investing in transformative technology companies that advance ABB’s vision of a more sustainable, electrified, and automated world. Edgecom was a winner in ABB’s 2024 Startup Challenge.
The International Energy Agency has stated that the world must double the pace of energy efficiency progress in the next decade to meet net zero targets. It highlights the key role digital energy management must play. Innovation teams at ABB’s Smart Power division will collaborate with Edgecom to develop new AI-enabled solutions to help customers in North America save energy and reduce costs.
Massimiliano Cifalitti, President of ABB’s Smart Power division, said: “Partnerships are key to ABB Electrification’s artificial intelligence strategy for energy management. Edgecom shows how gen AI can create business value from complex data sets with an easy-to-use interface. The company also has the scalability and interoperability ABB is looking for as we grow our AI ecosystem for energy management.”
Artificial intelligence can be a game-changer for energy management. ABB’s digitalised electrification solutions collect data from across a site’s power network; Edgecom’s AI Energy Copilot can turn complex dataset into energy saving opportunities. Adapting to the customer’s goals, the AI Energy Copilot suggests small adjustments to lower bills or smart ideas to reduce environmental footprint.
Behdad Bahrami, CEO and Co-Founder of Edgecom, said: “ABB’s commitment to our vision underscores the transformative impact we’re bringing to energy management. Together, we’re empowering large energy users to achieve significant cost savings and emissions reductions through innovative solutions that deliver real world cost savings and emissions reductions. As the energy transition accelerates, innovative partnerships like this are key to creating a more efficient and sustainable future for industries worldwide.”
Mehdi Parvizi, CTO and Co-Founder of Edgecom, added: “Generative AI is transforming energy management by enabling tailored strategies that unlock savings across energy-intensive assets and facilities. This technology optimizes asset performance, integrates operations with energy market programs and price tariffs, improves energy efficiency, and guides operator behavior toward more effective energy decisions.”
The investment in Edgecom brings the total portfolio of ABB Electrification Ventures to 15 companies, with investments totalling €80 million since 2021. It is part of ABB Ventures, which has invested more than $450 million in 70 startups across electrification and automation sectors since 2010.
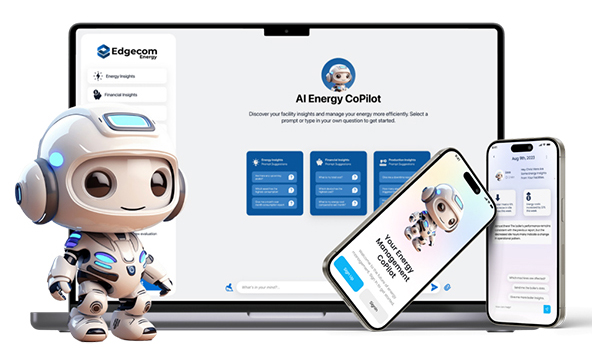
Edgecom Energy’s unique energy management platform uses generative AI to help users reduce power demand peaks
SourceABB
EMR Analysis
More information on ABB: See full profile on EMR Executive Services
More information on Morten Wierod (Chief Executive Officer and Member of the Group Executive Committee, ABB): See full profile on EMR Executive Services
More information on Timo Ihamuotila (Chief Financial Officer and Member of the Executive Committee, ABB): See full profile on EMR Executive Services
More information on Electrification Business Area by ABB: See the full profile on EMR Executive Services
More information on Giampiero Frisio (President, Electrification Business Area and Member of the Executive Committee, ABB): See full profile on EMR Executive Services
More information on Massimiliano Cifalitti (President, Smart Power Division, Electrification Business Area, ABB): See full profile on EMR Executive Services
More information on ABB Ventures by ABB: https://global.abb/group/en/technology/ventures + Strategic venture capital for breakthrough industrial technology startups
The ABB Group through its business-led venture capital investment framework, ABB Ventures, looks for breakthrough technology companies aligned with ABB’s goal to write the future of industrial electrification and automation. Since its formation in 2009, ABB Ventures has deployed around $450 million into 70 startups spanning a range of sectors including robotics, industrial IoT, AI/machine learning, energy transition, cybersecurity, sustainability, electric mobility, smart buildings, and distributed energy.
More information on Dr. Kurt Kaltenegger (Group Vice President, Head of ABB Technology Ventures, ABB): See full profile on EMR Executive Services
More information on ABB Electrification Ventures by ABB: https://global.abb/group/en/technology/ventures/electrification-ventures + As the world’s demand for electricity grows, we are electrifying the world in a safe, smart and sustainable way as a global technology leader in electrical distribution and management. We collaborate with customers and partners to enable energy efficiency and power a sustainable future for society with our products, solutions and digital technologies.
The investment in Edgecom brings the total portfolio of ABB Electrification Ventures to 15 companies, with investments totalling €80 million since 2021.
More information on Mads Møller (Head of Ventures, ABB Electrification Ventures, ABB): See full profile on EMR Executive Services
More information on Edgecom Energy (Strategic Investment by ABB): https://edgecom.ai/ + At Edgecom Energy, we empower large energy consumers to take control of their energy costs and reduce emissions by harnessing the power of IoT and AI Solutions. Our innovative technology helps companies optimize energy usage, save money, and contribute to a green, more sustainable future.
More information on Behdad Bahrami (Co-founder and Chief Executive Officer, Edgecom Energy): https://edgecom.ai/about-us/ + https://www.linkedin.com/in/bah-rami/
More information on Mehdi Parvizi (Co-founder and Chief Executive Officer, Edgecom Energy): https://www.linkedin.com/in/mehdi-parvizi-phd-2a5b5490/
More information on IEA (International Energy Agency): https://www.iea.org + The IEA is at the heart of global dialogue on energy, providing authoritative analysis, data, policy recommendations, and real-world solutions to help countries provide secure and sustainable energy for all.
The IEA was created in 1974 to help co-ordinate a collective response to major disruptions in the supply of oil. While oil security this remains a key aspect of our work, the IEA has evolved and expanded significantly since its foundation.
Taking an all-fuels, all-technology approach, the IEA recommends policies that enhance the reliability, affordability and sustainability of energy. It examines the full spectrum issues including renewables, oil, gas and coal supply and demand, energy efficiency, clean energy technologies, electricity systems and markets, access to energy, demand-side management, and much more.
Since 2015, the IEA has opened its doors to major emerging countries to expand its global impact, and deepen cooperation in energy security, data and statistics, energy policy analysis, energy efficiency, and the growing use of clean energy technologies.
More information on Dr. Fatih Birol (Executive Director, International Energy Agency): https://www.iea.org/contributors/dr-fatih-birol + https://www.linkedin.com/in/fatih-birol/
More information on Net Zero by 2050 by the United Nations: https://www.un.org/en/climatechange/net-zero-coalition + Put simply, net zero means cutting greenhouse gas emissions to as close to zero as possible, with any remaining emissions re-absorbed from the atmosphere, by oceans and forests for instance.
Currently, the Earth is already about 1.1°C warmer than it was in the late 1800s, and emissions continue to rise. To keep global warming to no more than 1.5°C – as called for in the Paris Agreement – emissions need to be reduced by 45% by 2030 and reach net zero by 2050.
More than 140 countries, including the biggest polluters – China, the United States, India and the European Union – have set a net-zero target, covering about 88% of global emissions. More than 9,000 companies, over 1000 cities, more than 1000 educational institutions, and over 600 financial institutions have joined the Race to Zero, pledging to take rigorous, immediate action to halve global emissions by 2030.
More information on Net Zero by 2050 by the Science Based Targets initiative (SBTi): https://sciencebasedtargets.org/net-zero + The SBTi’s Corporate Net-Zero Standard is the world’s only framework for corporate net-zero target setting in line with climate science. It includes the guidance, criteria, and recommendations companies need to set science-based net-zero targets consistent with limiting global temperature rise to 1.5°C.
UN vs. SBTi:
- UN targets nations, while SBTi focuses on companies. UN sets a broad goal, while SBTI provides a detailed framework for target setting.
- Both aim to achieve net zero emissions and limit warming to 1.5°C. The UN sets the overall direction, and SBTi helps businesses translate that goal into actionable plans.
Key components of the Corporate Net-Zero Standard:
- Near-term targets: Rapid, deep cuts to direct and indirect value-chain emissions must be the overarching priority for companies. Companies must set near-term science-based targets to roughly halve emission before 2030. This is the most effective, scientifically-sound way of limiting global temperature rise to 1.5°C.
- Long-term targets: Companies must set long-term science-based targets. Companies must cut all possible – usually more than 90% – of emissions before 2050.
- Neutralize residual emissions: After a company has achieved its long-term target and cut emissions by more than 90%, it must use permanent carbon removal and storage to counterbalance the final 10% or more of residual emissions that cannot be eliminated. A company is only considered to have reached net-zero when it has achieved its long-term science-based target and neutralized any residual emissions.
- Beyond Value Chain Mitigation (BVCM): Businesses should invest now in actions to reduce and remove emissions outside of their value chains in addition to near- and long-term science-based targets.
EMR Additional Notes:
- AI – Artificial Intelligence:
- https://searchenterpriseai.techtarget.com/definition/AI-Artificial-Intelligence +
- Artificial intelligence is the simulation of human intelligence processes by machines, especially computer systems.
- As the hype around AI has accelerated, vendors have been scrambling to promote how their products and services use AI. Often what they refer to as AI is simply one component of AI, such as machine learning. AI requires a foundation of specialized hardware and software for writing and training machine learning algorithms. No one programming language is synonymous with AI, but well a few, including Python, R and Java, are popular.
- In general, AI systems work by ingesting large amounts of labeled training data, analyzing the data for correlations and patterns, and using these patterns to make predictions about future states. In this way, a chatbot that is fed examples of text chats can learn to produce lifelike exchanges with people, or an image recognition tool can learn to identify and describe objects in images by reviewing millions of examples.
- AI programming focuses on three cognitive skills: learning, reasoning and self-correction.
- What are the 4 types of artificial intelligence?
- Type 1: Reactive machines. These AI systems have no memory and are task specific. An example is Deep Blue, the IBM chess program that beat Garry Kasparov in the 1990s. Deep Blue can identify pieces on the chessboard and make predictions, but because it has no memory, it cannot use past experiences to inform future ones.
- Type 2: Limited memory. These AI systems have memory, so they can use past experiences to inform future decisions. Some of the decision-making functions in self-driving cars are designed this way.
- Type 3: Theory of mind. Theory of mind is a psychology term. When applied to AI, it means that the system would have the social intelligence to understand emotions. This type of AI will be able to infer human intentions and predict behavior, a necessary skill for AI systems to become integral members of human teams.
- Type 4: Self-awareness. In this category, AI systems have a sense of self, which gives them consciousness. Machines with self-awareness understand their own current state. This type of AI does not yet exist.
- Machine Learning (ML):
- Developed to mimic human intelligence, it lets the machines learn independently by ingesting vast amounts of data, statistics formulas and detecting patterns.
- ML allows software applications to become more accurate at predicting outcomes without being explicitly programmed to do so.
- ML algorithms use historical data as input to predict new output values.
- Recommendation engines are a common use case for ML. Other uses include fraud detection, spam filtering, business process automation (BPA) and predictive maintenance.
- Classical ML is often categorized by how an algorithm learns to become more accurate in its predictions. There are four basic approaches: supervised learning, unsupervised learning, semi-supervised learning and reinforcement learning.
- Deep Learning (DL):
- Subset of machine learning, Deep Learning enabled much smarter results than were originally possible with ML. Face recognition is a good example.
- DL makes use of layers of information processing, each gradually learning more and more complex representations of data. The early layers may learn about colors, the next ones about shapes, the following about combinations of those shapes, and finally actual objects. DL demonstrated a breakthrough in object recognition.
- DL is currently the most sophisticated AI architecture we have developed.
- Computer Vision (CV):
- Computer vision is a field of artificial intelligence that enables computers and systems to derive meaningful information from digital images, videos and other visual inputs — and take actions or make recommendations based on that information.
- The most well-known case of this today is Google’s Translate, which can take an image of anything — from menus to signboards — and convert it into text that the program then translates into the user’s native language.
- Machine Vision (MV):
- Machine Vision is the ability of a computer to see; it employs one or more video cameras, analog-to-digital conversion and digital signal processing. The resulting data goes to a computer or robot controller. Machine Vision is similar in complexity to Voice Recognition.
- MV uses the latest AI technologies to give industrial equipment the ability to see and analyze tasks in smart manufacturing, quality control, and worker safety.
- Computer Vision systems can gain valuable information from images, videos, and other visuals, whereas Machine Vision systems rely on the image captured by the system’s camera. Another difference is that Computer Vision systems are commonly used to extract and use as much data as possible about an object.
- Generative AI (GenAI):
- Generative AI technology generates outputs based on some kind of input – often a prompt supplied by a person. Some GenAI tools work in one medium, such as turning text inputs into text outputs, for example. With the public release of ChatGPT in late November 2022, the world at large was introduced to an AI app capable of creating text that sounded more authentic and less artificial than any previous generation of computer-crafted text.
- https://searchenterpriseai.techtarget.com/definition/AI-Artificial-Intelligence +
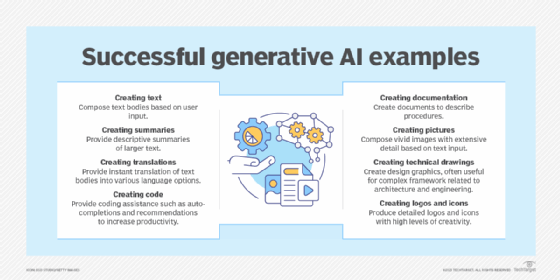
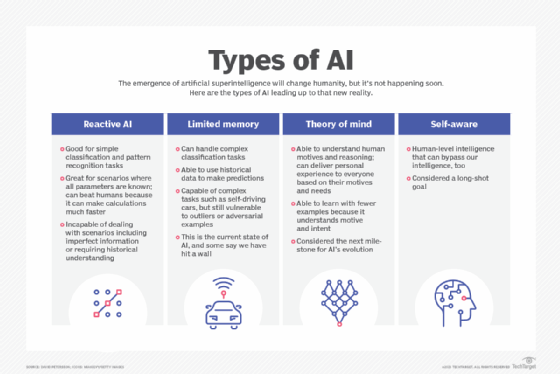
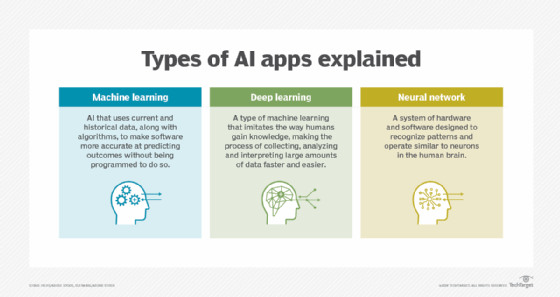
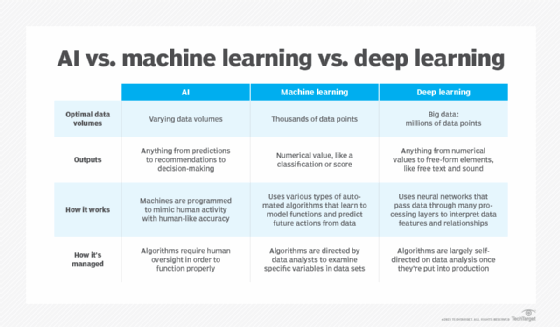
- Edge AI Technology:
- Edge artificial intelligence refers to the deployment of AI algorithms and AI models directly on local edge devices such as sensors or Internet of Things (IoT) devices, which enables real-time data processing and analysis without constant reliance on cloud infrastructure.
- Simply stated, edge AI, or “AI on the edge“, refers to the combination of edge computing and artificial intelligence to execute machine learning tasks directly on interconnected edge devices. Edge computing allows for data to be stored close to the device location, and AI algorithms enable the data to be processed right on the network edge, with or without an internet connection. This facilitates the processing of data within milliseconds, providing real-time feedback.
- Self-driving cars, wearable devices, security cameras, and smart home appliances are among the technologies that leverage edge AI capabilities to promptly deliver users with real-time information when it is most essential.
- Multimodal Intelligence and Agents:
- Subset of artificial intelligence that integrates information from various modalities, such as text, images, audio, and video, to build more accurate and comprehensive AI models.
- Multimodal capabilities allows to interact with users in a more natural and intuitive way. It can see, hear and speak, which means that users can provide input and receive responses in a variety of ways.
- An AI agent is a computational entity designed to act independently. It performs specific tasks autonomously by making decisions based on its environment, inputs, and a predefined goal. What separates an AI agent from an AI model is the ability to act. There are many different kinds of agents such as reactive agents and proactive agents. Agents can also act in fixed and dynamic environments. Additionally, more sophisticated applications of agents involve utilizing agents to handle data in various formats, known as multimodal agents and deploying multiple agents to tackle complex problems.
- Small Language Models (SLM) and Large Language Models (LLM):
- Small language models (SLMs) are artificial intelligence (AI) models capable of processing, understanding and generating natural language content. As their name implies, SLMs are smaller in scale and scope than large language models (LLMs).
- LLM means large language model—a type of machine learning/deep learning model that can perform a variety of natural language processing (NLP) and analysis tasks, including translating, classifying, and generating text; answering questions in a conversational manner; and identifying data patterns.
- For example, virtual assistants like Siri, Alexa, or Google Assistant use LLMs to process natural language queries and provide useful information or execute tasks such as setting reminders or controlling smart home devices.