Signify – Signify #1 Lighting company in European patent filings in 2024
- Ranks second among Dutch companies in the list, filing for 742 patents
- Signify in 23rd placement out of all companies that filed patents with the EPO
Eindhoven, the Netherlands – Signify (Euronext: LIGHT), the world leader in lighting, in 2024 ranks as the number one Lighting company in patent filings at the European Patent Office (EPO), illustrating the company’s commitment to responsible innovation, energy-efficient LED lighting technology, and advancements in IoT and generative AI. Signify filed a total of 742 patents, ranking 23 out of all companies that filed patents with the EPO.
This year’s EPO ranking reflects our unwavering commitment to build a robust portfolio of lighting innovations that revolutionize our industry. Our technological advances have led to leaps forward in energy efficiency, extending product lifespans and enhancing well-being and experiences in homes, workplaces and communities around the world”
Olivia Qiu,
Chief Innovation Officer, Signify
In 2024, Signify filed a growing number of patents focused on enhancing the efficiency and quality of lighting. Many new innovations are driven by generative AI, which has enabled Signify to improve its smart controls, sensors and other functionality to deliver superior lighting solutions. For instance, the AI understands the specific needs of users and automatically controls the lighting based on that, while improving the interfacing with non-lighting systems.
“Despite political and economic uncertainties, European companies and inventors filed more patents last year, underlining their technological prowess and their continued investment in R&D,” said EPO President António Campinos.
The EPO, headquartered in Munich, enables inventors to obtain high-quality patent protection in up to 45 countries, covering a market of some 700 million people. Last year, it received a total of 199,264 applications.
For detailed statistics and supporting materials produced by the European Patent Office, see the EPO’s Annual Report at: www.epo.org/en/about-us/statistics/patent-index-2024.
SourceSignify
EMR Analysis
More information on Signify: See the full profile on EMR Executive Services
More information on Eric Rondolat (Chair of the Board of Management and Chief Executive Officer, Signify till April 25, 2025): See the full profile on EMR Executive Services
More information on Olivia Qiu (Chief Innovation Officer, Signify): See the full profile on EMR Executive Services
More information on the European Patent Office: https://www.epo.org/en + The European Patent Office (EPO) examines European patent applications, enabling inventors, researchers and companies from around the world to obtain protection for their inventions in up to 45 countries through a centralised and uniform procedure that requires just one application.
More information on António Campinos (President, EPO): https://www.epo.org/en/about-us/at-a-glance
EMR Additional Notes:
- LED:
- LED stands for light emitting diode. LED lighting products produce light up to 90% more efficiently than incandescent light bulbs. How do they work? An electrical current passes through a microchip, which illuminates the tiny light sources we call LEDs and the result is visible light.
- A light-emitting diode is a semiconductor light source that emits light when current flows through it. Electrons in the semiconductor recombine with electron holes, releasing energy in the form of photons.
- LED vs. Halogen:
- Halogen bulbs, while lasting longer than incandescent bulbs, only last up to 2,000 hours. In contrast, LED bulbs can last up to 25,000 hours, and LED tubes are rated for up to 50,000 hours. LED bulbs can use as much as 80% percent less energy than halogen bulbs.
- There’s obviously a clear winner when it comes to LED vs halogen lighting. LED lights are more energy-efficient, have a longer lifespan, and offer more choices in color temperature. They do cost a little more, but their extremely long lifespan easily offsets the higher upfront cost.
- microLED:
- Compared to widespread LCD technology, microLED displays offer better contrast, response times, and energy efficiency. They are also capable of high speed modulation, and have been proposed for chip-to-chip interconnect applications.
- MicroLED prototype displays have been shown to offer up to 10 times more brightness than the best OLED panel while being significantly more power efficient, making them an exciting new technology in the world of displays.
- OLED:
- An Organic Light-Emitting Diode is a solid-state device consisting of a thin, carbon-based semiconductor layer that emits light when electricity is applied by adjacent electrodes. In order for light to escape from the device, at least one of the electrodes must be transparent.
- OLED devices (television screens, computer monitors, and portable systems such as smartphones …) use LED technology and use an organic material as a light emitting layer. Organic LEDs can produce high quality displays with high contrasts, high viewing angles and true blacks. Some say that OLEDs produce the world’s best display panels.
- AI – Artificial Intelligence:
- Artificial intelligence is the simulation of human intelligence processes by machines, especially computer systems.
- As the hype around AI has accelerated, vendors have been scrambling to promote how their products and services use AI. Often what they refer to as AI is simply one component of AI, such as machine learning. AI requires a foundation of specialized hardware and software for writing and training machine learning algorithms. No one programming language is synonymous with AI, but well a few, including Python, R and Java, are popular.
- In general, AI systems work by ingesting large amounts of labeled training data, analyzing the data for correlations and patterns, and using these patterns to make predictions about future states. In this way, a chatbot that is fed examples of text chats can learn to produce lifelike exchanges with people, or an image recognition tool can learn to identify and describe objects in images by reviewing millions of examples.
- AI programming focuses on three cognitive skills: learning, reasoning and self-correction.
- What are the 4 types of artificial intelligence?
- Type 1: Reactive machines. These AI systems have no memory and are task specific. An example is Deep Blue, the IBM chess program that beat Garry Kasparov in the 1990s. Deep Blue can identify pieces on the chessboard and make predictions, but because it has no memory, it cannot use past experiences to inform future ones.
- Type 2: Limited memory. These AI systems have memory, so they can use past experiences to inform future decisions. Some of the decision-making functions in self-driving cars are designed this way.
- Type 3: Theory of mind. Theory of mind is a psychology term. When applied to AI, it means that the system would have the social intelligence to understand emotions. This type of AI will be able to infer human intentions and predict behavior, a necessary skill for AI systems to become integral members of human teams.
- Type 4: Self-awareness. In this category, AI systems have a sense of self, which gives them consciousness. Machines with self-awareness understand their own current state. This type of AI does not yet exist.
- Machine Learning (ML):
- Developed to mimic human intelligence, it lets the machines learn independently by ingesting vast amounts of data, statistics formulas and detecting patterns.
- ML allows software applications to become more accurate at predicting outcomes without being explicitly programmed to do so.
- ML algorithms use historical data as input to predict new output values.
- Recommendation engines are a common use case for ML. Other uses include fraud detection, spam filtering, business process automation (BPA) and predictive maintenance.
- Classical ML is often categorized by how an algorithm learns to become more accurate in its predictions. There are four basic approaches: supervised learning, unsupervised learning, semi-supervised learning and reinforcement learning.
- Deep Learning (DL):
- Subset of machine learning, Deep Learning enabled much smarter results than were originally possible with ML. Face recognition is a good example.
- DL makes use of layers of information processing, each gradually learning more and more complex representations of data. The early layers may learn about colors, the next ones about shapes, the following about combinations of those shapes, and finally actual objects. DL demonstrated a breakthrough in object recognition.
- DL is currently the most sophisticated AI architecture we have developed.
- Computer Vision (CV):
- Computer vision is a field of artificial intelligence that enables computers and systems to derive meaningful information from digital images, videos and other visual inputs — and take actions or make recommendations based on that information.
- The most well-known case of this today is Google’s Translate, which can take an image of anything — from menus to signboards — and convert it into text that the program then translates into the user’s native language.
- Machine Vision (MV):
- Machine Vision is the ability of a computer to see; it employs one or more video cameras, analog-to-digital conversion and digital signal processing. The resulting data goes to a computer or robot controller. Machine Vision is similar in complexity to Voice Recognition.
- MV uses the latest AI technologies to give industrial equipment the ability to see and analyze tasks in smart manufacturing, quality control, and worker safety.
- Computer Vision systems can gain valuable information from images, videos, and other visuals, whereas Machine Vision systems rely on the image captured by the system’s camera. Another difference is that Computer Vision systems are commonly used to extract and use as much data as possible about an object.
- Generative AI (GenAI):
- Generative AI technology generates outputs based on some kind of input – often a prompt supplied by a person. Some GenAI tools work in one medium, such as turning text inputs into text outputs, for example. With the public release of ChatGPT in late November 2022, the world at large was introduced to an AI app capable of creating text that sounded more authentic and less artificial than any previous generation of computer-crafted text.
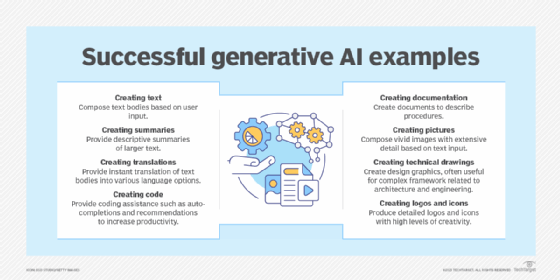
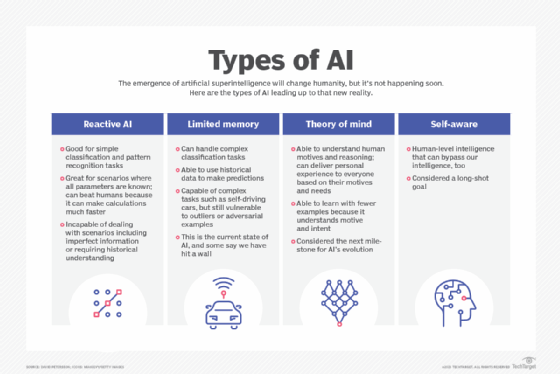
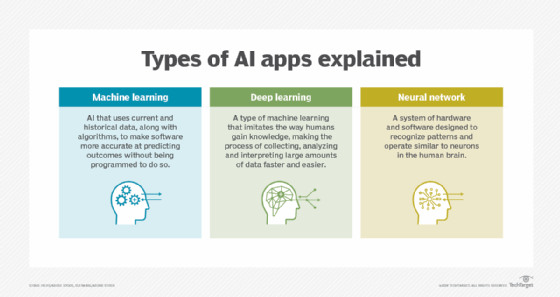
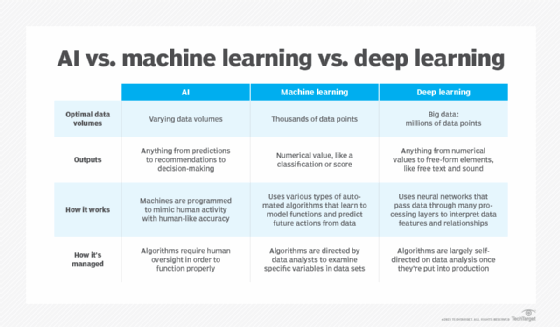
- Edge AI Technology:
- Edge artificial intelligence refers to the deployment of AI algorithms and AI models directly on local edge devices such as sensors or Internet of Things (IoT) devices, which enables real-time data processing and analysis without constant reliance on cloud infrastructure.
- Simply stated, edge AI, or “AI on the edge“, refers to the combination of edge computing and artificial intelligence to execute machine learning tasks directly on interconnected edge devices. Edge computing allows for data to be stored close to the device location, and AI algorithms enable the data to be processed right on the network edge, with or without an internet connection. This facilitates the processing of data within milliseconds, providing real-time feedback.
- Self-driving cars, wearable devices, security cameras, and smart home appliances are among the technologies that leverage edge AI capabilities to promptly deliver users with real-time information when it is most essential.
- Multimodal Intelligence and Agents:
- Subset of artificial intelligence that integrates information from various modalities, such as text, images, audio, and video, to build more accurate and comprehensive AI models.
- Multimodal capabilities allows to interact with users in a more natural and intuitive way. It can see, hear and speak, which means that users can provide input and receive responses in a variety of ways.
- An AI agent is a computational entity designed to act independently. It performs specific tasks autonomously by making decisions based on its environment, inputs, and a predefined goal. What separates an AI agent from an AI model is the ability to act. There are many different kinds of agents such as reactive agents and proactive agents. Agents can also act in fixed and dynamic environments. Additionally, more sophisticated applications of agents involve utilizing agents to handle data in various formats, known as multimodal agents and deploying multiple agents to tackle complex problems.
- Small Language Models (SLM) and Large Language Models (LLM):
- Small language models (SLMs) are artificial intelligence (AI) models capable of processing, understanding and generating natural language content. As their name implies, SLMs are smaller in scale and scope than large language models (LLMs).
- LLM means large language model—a type of machine learning/deep learning model that can perform a variety of natural language processing (NLP) and analysis tasks, including translating, classifying, and generating text; answering questions in a conversational manner; and identifying data patterns.
- For example, virtual assistants like Siri, Alexa, or Google Assistant use LLMs to process natural language queries and provide useful information or execute tasks such as setting reminders or controlling smart home devices.
- Agentic AI:
- Agentic AI is an artificial intelligence system that can accomplish a specific goal with limited supervision. It consists of AI agents—machine learning models that mimic human decision-making to solve problems in real time. In a multiagent system, each agent performs a specific subtask required to reach the goal and their efforts are coordinated through AI orchestration.
- Unlike traditional AI models, which operate within predefined constraints and require human intervention, agentic AI exhibits autonomy, goal-driven behavior and adaptability. The term “agentic” refers to these models’ agency, or, their capacity to act independently and purposefully.
- Agentic AI builds on generative AI (gen AI) techniques by using large language models (LLMs) to function in dynamic environments. While generative models focus on creating content based on learned patterns, agentic AI extends this capability by applying generative outputs toward specific goals.
- IoT (Internet of Things):
- The Internet of Things (IoT) refers to a system of interrelated, internet-connected objects that are able to collect and transfer data over a wireless network without human intervention.
- Describes the network of physical objects—“things”—that are embedded with sensors, software, and other technologies for the purpose of connecting and exchanging data with other devices and systems over the internet.
- The Most Popular IoT Devices are:
- Smart watches are the most popular IoT devices. …
- Gaming consoles. …
- Smart TV sets and content streaming devices. …
- Voice control devices. …
- Printers. …
- Cameras. …
- Lighting appliances. …
- Smart thermostats.
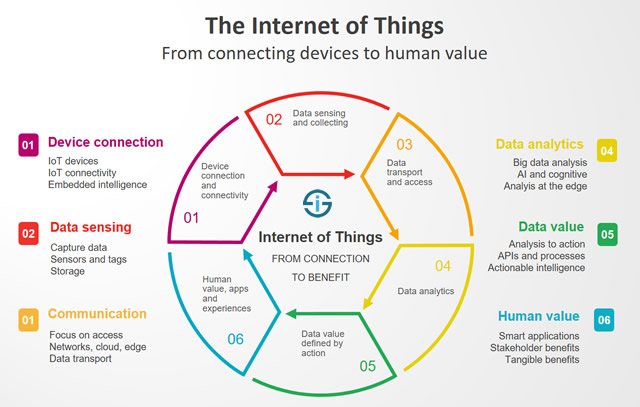
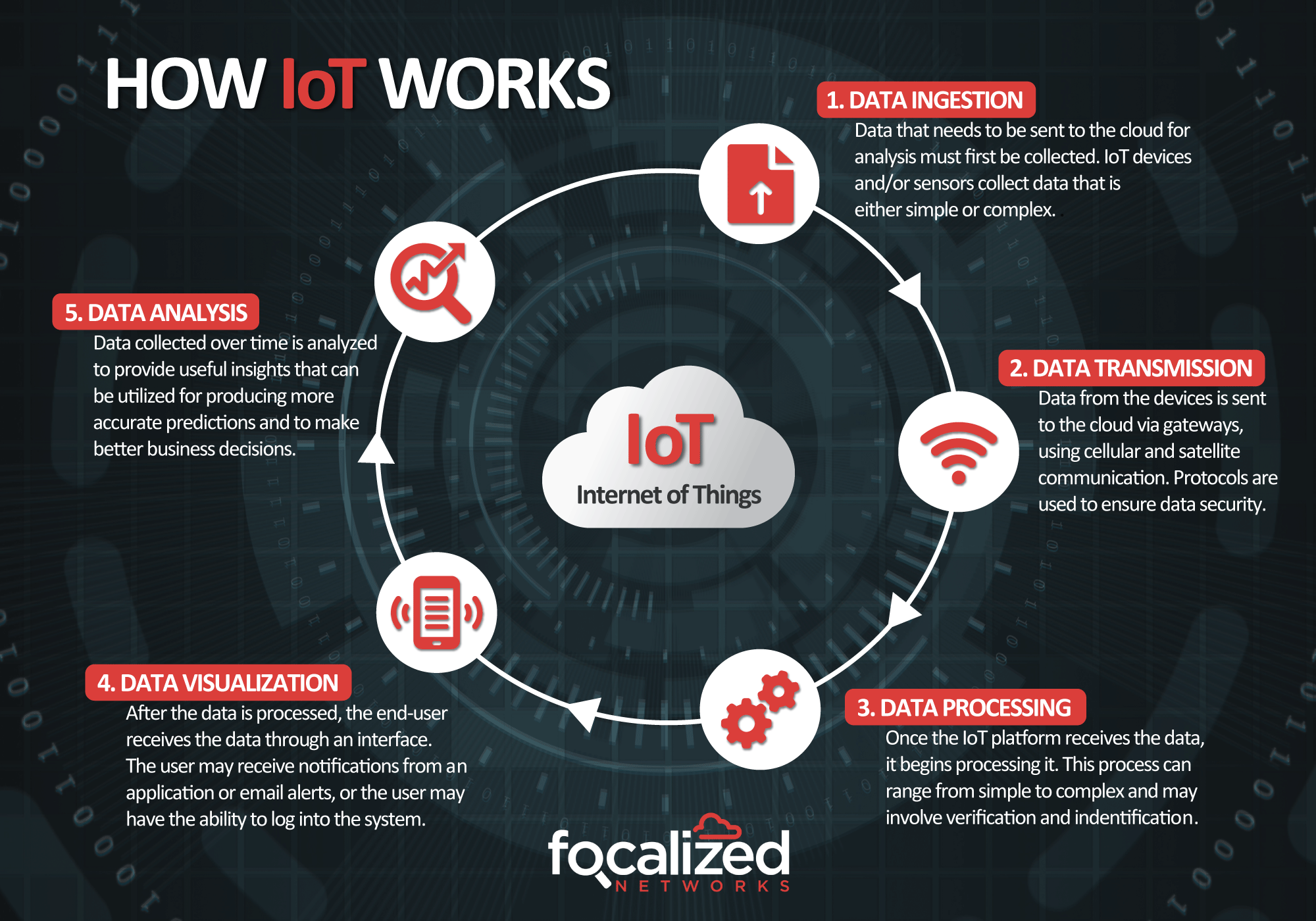
- IIoT (Industrial IoT):
- Industrial IoT (IIoT) involves collecting and analyzing sensor-generated data to support equipment monitoring and maintenance, production process analytics and control, and more. In manufacturing IT since 1989, ScienceSoft offers IIoT consulting and development to create secure IIoT solutions.
- AIoT (Artificial Intelligence of Things):
- Relatively new term and has recently become a hot topic which combines two of the hottest acronyms, AI (Artificial Intelligence) and IoT (Internet of Things).
- AIoT is transformational and reciprocally beneficial for both types of technology, as AI adds value to IoT through machine learning capabilities and improved decision-making processes, while IoT adds value to AI through connectivity, signalling, and data exchange.
- Aim: achieve more efficient IoT operations, improve human-machine interactions and enhance data management and analytics.
- xIoT (xTended Internet of Things):
- xIoT refers to the “xTended” Internet of Things. This xTended IoT category spans Enterprise IoT devices (cameras, printers, and door controllers), OT devices (like PLCs, HMI’s, and robotics) and Network devices (like switches, WiFi routers, and NAS).