ABB – ABB plans to spin off its robotics division as a separately listed company
- A strong performer in its industry at the core of secular and future automation trends
- Plan for a listing in the second quarter of 2026
- Foreseen to be distributed to ABB’s shareholders as a dividend in-kind in proportion to existing shareholding
ABB announced today that it will launch a process to propose to its Annual General Meeting 2026 to decide on a 100 percent spin-off of its Robotics division. The intention is for the business to start trading as a separately listed company during the second quarter of 2026.
The board believes listing ABB Robotics as a separate company will optimize both companies’ ability to create customer value, grow and attract talent. Both companies will benefit from a more focused governance and capital allocation. ABB will continue to focus on its long-term strategy, building on its leading positions in electrification and automation.
ABB Chairman Peter Voser
ABB Robotics is a technology leader and provides intelligent automation solutions to help its global customer base achieve improved productivity, flexibility and simplicity to solve operational challenges including labor shortages and the need to operate more sustainably. Customer value is created through the differentiated offering of the broadest robotics platforms, including Autonomous Mobile Robots, software and AI combined with proven domain expertise to a broad range of traditional and new industry segments. More than 80 percent of the offering is software/AI enabled.
ABB Robotics is a leader in its industry and there are limited business and technology synergies between the ABB Robotics business and the remainder of ABB divisions, with different demand and market characteristics. We believe this change will support value creation in both the ABB Group and in the separately listed pure play robotics business.
ABB CEO Morten Wierod
The ABB Robotics division is a strong performer amongst its peers. Under the ABB Way decentralized operating model ABB Robotics has proven its double-digit margin resilience in most quarters since 2019. The market has seemingly stabilized – supporting the divisional order growth – after what has been an unusually volatile market situation which has included the normalization of order patterns after the period of pre-buys when the supply chain was strained. The company will be listed with a strong capital structure, is well invested with a solid cash flow profile and operates through its local-for-local set-up with regional manufacturing hubs in Europe (Sweden), Asia (China) and the Americas (United States).
The ABB Robotics division has approximately 7,000 employees. With 2024 revenues of $2.3 billion it represented about 7 percent of ABB Group revenues and had an Operational EBITA margin of 12.1 percent.
If shareholders decide in favor of the proposal, the spin-off is planned to be done through a share distribution, whereby ABB Ltd.’s shareholders will receive shares in the company to be listed (working name “ABB Robotics”) as a dividend in-kind in proportion to their existing shareholding.
As from the first quarter of 2026, the Machine Automation division, which together with ABB Robotics currently forms the Robotics & Discrete Automation business area, will become a part of the Process Automation business area, where the customer value creation ability in divisions will benefit from technology synergies for software and control technologies, for example towards hybrid industries. The Machine Automation business holds a leading position in the high-end segment for solutions based on PLCs, IPCs, servo motion, industrial transport systems and vision and software. The strategy and business priorities remain the responsibility of the divisional management team, under the ABB Way operating model.
Important notice about forward-looking information
This press release includes forward-looking information and statements as well as other statements concerning the planned spin-off of our robotics business. These statements are based on current expectations, estimates and projections about the factors that may affect our future performance, including the economic conditions of the regions and industries that are major markets for ABB. These expectations, estimates and projections are generally identifiable by statements containing words such as “believes”, ”foresees”, “expects”, “intends”, “plans”, or similar expressions. However, there are many risks and uncertainties, many of which are beyond our control, that could impact the spin-off, including business risks associated with the volatile global economic environment and political conditions. Although ABB Ltd believes that its expectations reflected in any such forward looking statement are based upon reasonable assumptions, it can give no assurance that those expectations will be achieved.
SourceABB
EMR Analysis
More information on ABB: See full profile on EMR Executive Services
More information on Peter Voser (Chairman of the Board of Directors, ABB Ltd + Chairman of the Governance and Nomination Committee, ABB Ltd): See full profile on EMR Executive Services
More information on Morten Wierod (Chief Executive Officer and Member of the Group Executive Committee, ABB): See full profile on EMR Executive Services
More information on Timo Ihamuotila (Chief Financial Officer and Member of the Executive Committee, ABB): See full profile on EMR Executive Services
More information on Robotics & Discrete Automation Business Area by ABB: See full profile on EMR Executive Services
More information on Sami Atiya (President, Robotics & Discrete Automation Business Area and Member of the Executive Committee, ABB): See full profile on EMR Executive Services
More information on Robotics Division by Robotics & Discrete Automation Business Area by ABB: See full profile on EMR Executive Services
More information on Marc Segura (President, ABB Robotics Division, Robotics & Discrete Automation Business Area, ABB): See full profile on EMR Executive Services
More information on Machine Automation Division by Robotics & Discrete Automation Business Area by ABB: See full profile on EMR Executive Services
More information on Jörg Theis (President, Machine Automation Division, Robotics & Discrete Automation Business Area, ABB + Chief Executive Officer, B&R Automation): See full profile on EMR Executive Services
More information on the ABB Way: See full profile on EMR Executive Services
More information on Process Automation Business Area by ABB: See the full profile on EMR Executive Services
More information on Peter Terwiesch (President, Process Automation Business Area and Member of the Executive Committee, ABB): See full profile on EMR Executive Services
EMR Additional Notes:
- Cobots (Collaborative Robots):
- A collaborative robot, also known as a cobot, is a robot that is capable of learning multiple tasks so it can assist human beings. In contrast, autonomous robots are hard-coded to repeatedly perform one task, work independently and remain stationary.
- Intended to work hand-in-hand with employees. These machines focus more on repetitive tasks, such as inspection and picking, to help workers focus more on tasks that require problem-solving skills.
- A robot is an autonomous machine that performs a task without human control. A cobot is an artificially intelligent robot that performs tasks in collaboration with human workers.
- According to ISO 10218 part 1 and part 2, there are four main types of collaborative robots: safety monitored stop, speed and separation, power and force limiting, and hand guiding.
- Autonomous Mobile Robot (AMR):
- Any robot that can understand and move through its environment without being overseen directly by an operator or on a fixed predetermined path. AMRs have an array of sophisticated sensors that enable them to understand and interpret their environment, which helps them to perform their task in the most efficient manner and path possible, navigating around fixed obstructions (building, racks, work stations, etc.) and variable obstructions (such as people, lift trucks, and debris). Though similar in many ways to automated guided vehicles (AGVs), AMRs differ in a number of important ways. The greatest of these differences is flexibility: AGVs must follow much more rigid, preset routes than AMRs. Autonomous mobile robots find the most efficient route to achieve each task, and are designed to work collaboratively with operators such as picking and sortation operations, whereas AGVs typically do not.
- Automated Guided Vehicles (AGV):
- An AGV system, or automated guided vehicle system, otherwise known as an automatic guided vehicle, autonomous guided vehicle or even automatic guided cart, is a system which follows a predestined path around a facility.
- Three types of AGVs are towing, fork trucks, and heavy load carriers. Each is designed to perform repetitive actions such as delivering raw materials, keep loads stable, and complete simple tasks.
- The main difference between an AGV and an AMR is that AMRs use free navigation by means of lasers, while AGVs are located with fixed elements: magnetic tapes, magnets, beacons, etc. So, to be effective, they must have a predictable route.
- Autonomous Case-handling Robots (ACR):
- Autonomous Case-handling Robot (ACR) systems are highly efficient “Goods to Person” solutions designed for totes & cartons transportation and process optimization, providing efficient, intelligent, flexible, and cost-effective warehouse automation solutions through robotics technology.
- Software vs. Hardware vs. Firmware:
- Hardware is physical: It’s “real,” sometimes breaks, and eventually wears out.
- Since hardware is part of the “real” world, it all eventually wears out. Being a physical thing, it’s also possible to break it, drown it, overheat it, and otherwise expose it to the elements.
- Here are some examples of hardware:
- Smartphone
- Tablet
- Laptop
- Desktop computer
- Printer
- Flash drive
- Router
- Software is virtual: It can be copied, changed, and destroyed.
- Software is everything about your computer that isn’t hardware.
- Here are some examples of software:
- Operating systems like Windows 11 or iOS
- Web browsers
- Antivirus tools
- Adobe Photoshop
- Mobile apps
- Firmware is virtual: It’s software specifically designed for a piece of hardware
- While not as common a term as hardware or software, firmware is everywhere—on your smartphone, your PC’s motherboard, your camera, your headphones, and even your TV remote control.
- Firmware is just a special kind of software that serves a very narrow purpose for a piece of hardware. While you might install and uninstall software on your computer or smartphone on a regular basis, you might only rarely, if ever, update the firmware on a device, and you’d probably only do so if asked by the manufacturer, probably to fix a problem.
- Hardware is physical: It’s “real,” sometimes breaks, and eventually wears out.
- AI – Artificial Intelligence:
- Artificial intelligence is the simulation of human intelligence processes by machines, especially computer systems.
- As the hype around AI has accelerated, vendors have been scrambling to promote how their products and services use AI. Often what they refer to as AI is simply one component of AI, such as machine learning. AI requires a foundation of specialized hardware and software for writing and training machine learning algorithms. No one programming language is synonymous with AI, but well a few, including Python, R and Java, are popular.
- In general, AI systems work by ingesting large amounts of labeled training data, analyzing the data for correlations and patterns, and using these patterns to make predictions about future states. In this way, a chatbot that is fed examples of text chats can learn to produce lifelike exchanges with people, or an image recognition tool can learn to identify and describe objects in images by reviewing millions of examples.
- AI programming focuses on three cognitive skills: learning, reasoning and self-correction.
- What are the 4 types of artificial intelligence?
- Type 1: Reactive machines. These AI systems have no memory and are task specific. An example is Deep Blue, the IBM chess program that beat Garry Kasparov in the 1990s. Deep Blue can identify pieces on the chessboard and make predictions, but because it has no memory, it cannot use past experiences to inform future ones.
- Type 2: Limited memory. These AI systems have memory, so they can use past experiences to inform future decisions. Some of the decision-making functions in self-driving cars are designed this way.
- Type 3: Theory of mind. Theory of mind is a psychology term. When applied to AI, it means that the system would have the social intelligence to understand emotions. This type of AI will be able to infer human intentions and predict behavior, a necessary skill for AI systems to become integral members of human teams.
- Type 4: Self-awareness. In this category, AI systems have a sense of self, which gives them consciousness. Machines with self-awareness understand their own current state. This type of AI does not yet exist.
- Machine Learning (ML):
- Developed to mimic human intelligence, it lets the machines learn independently by ingesting vast amounts of data, statistics formulas and detecting patterns.
- ML allows software applications to become more accurate at predicting outcomes without being explicitly programmed to do so.
- ML algorithms use historical data as input to predict new output values.
- Recommendation engines are a common use case for ML. Other uses include fraud detection, spam filtering, business process automation (BPA) and predictive maintenance.
- Classical ML is often categorized by how an algorithm learns to become more accurate in its predictions. There are four basic approaches: supervised learning, unsupervised learning, semi-supervised learning and reinforcement learning.
- Deep Learning (DL):
- Subset of machine learning, Deep Learning enabled much smarter results than were originally possible with ML. Face recognition is a good example.
- DL makes use of layers of information processing, each gradually learning more and more complex representations of data. The early layers may learn about colors, the next ones about shapes, the following about combinations of those shapes, and finally actual objects. DL demonstrated a breakthrough in object recognition.
- DL is currently the most sophisticated AI architecture we have developed.
- Computer Vision (CV):
- Computer vision is a field of artificial intelligence that enables computers and systems to derive meaningful information from digital images, videos and other visual inputs — and take actions or make recommendations based on that information.
- The most well-known case of this today is Google’s Translate, which can take an image of anything — from menus to signboards — and convert it into text that the program then translates into the user’s native language.
- Machine Vision (MV):
- Machine Vision is the ability of a computer to see; it employs one or more video cameras, analog-to-digital conversion and digital signal processing. The resulting data goes to a computer or robot controller. Machine Vision is similar in complexity to Voice Recognition.
- MV uses the latest AI technologies to give industrial equipment the ability to see and analyze tasks in smart manufacturing, quality control, and worker safety.
- Computer Vision systems can gain valuable information from images, videos, and other visuals, whereas Machine Vision systems rely on the image captured by the system’s camera. Another difference is that Computer Vision systems are commonly used to extract and use as much data as possible about an object.
- Generative AI (GenAI):
- Generative AI technology generates outputs based on some kind of input – often a prompt supplied by a person. Some GenAI tools work in one medium, such as turning text inputs into text outputs, for example. With the public release of ChatGPT in late November 2022, the world at large was introduced to an AI app capable of creating text that sounded more authentic and less artificial than any previous generation of computer-crafted text.
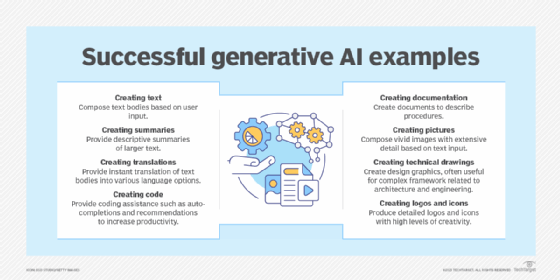
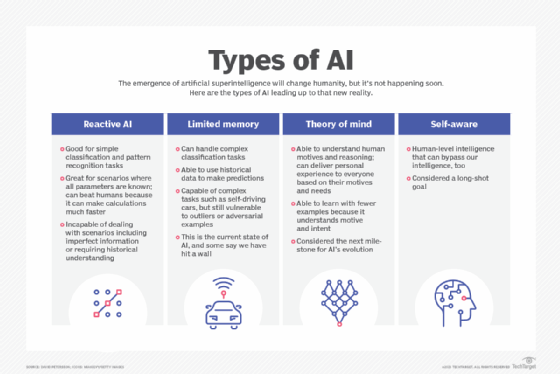
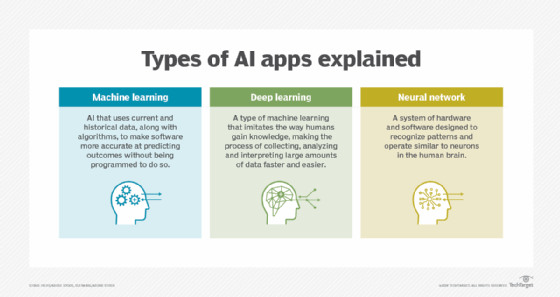
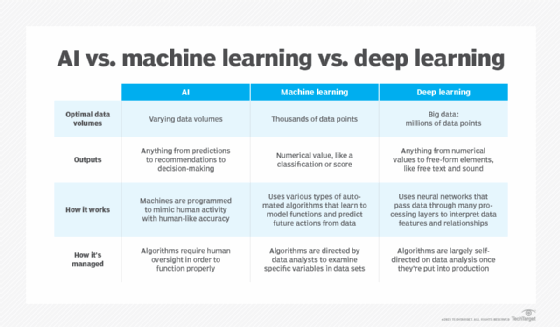
- Edge AI Technology:
- Edge artificial intelligence refers to the deployment of AI algorithms and AI models directly on local edge devices such as sensors or Internet of Things (IoT) devices, which enables real-time data processing and analysis without constant reliance on cloud infrastructure.
- Simply stated, edge AI, or “AI on the edge“, refers to the combination of edge computing and artificial intelligence to execute machine learning tasks directly on interconnected edge devices. Edge computing allows for data to be stored close to the device location, and AI algorithms enable the data to be processed right on the network edge, with or without an internet connection. This facilitates the processing of data within milliseconds, providing real-time feedback.
- Self-driving cars, wearable devices, security cameras, and smart home appliances are among the technologies that leverage edge AI capabilities to promptly deliver users with real-time information when it is most essential.
- Multimodal Intelligence and Agents:
- Subset of artificial intelligence that integrates information from various modalities, such as text, images, audio, and video, to build more accurate and comprehensive AI models.
- Multimodal capabilities allows to interact with users in a more natural and intuitive way. It can see, hear and speak, which means that users can provide input and receive responses in a variety of ways.
- An AI agent is a computational entity designed to act independently. It performs specific tasks autonomously by making decisions based on its environment, inputs, and a predefined goal. What separates an AI agent from an AI model is the ability to act. There are many different kinds of agents such as reactive agents and proactive agents. Agents can also act in fixed and dynamic environments. Additionally, more sophisticated applications of agents involve utilizing agents to handle data in various formats, known as multimodal agents and deploying multiple agents to tackle complex problems.
- Small Language Models (SLM) and Large Language Models (LLM):
- Small language models (SLMs) are artificial intelligence (AI) models capable of processing, understanding and generating natural language content. As their name implies, SLMs are smaller in scale and scope than large language models (LLMs).
- LLM means large language model—a type of machine learning/deep learning model that can perform a variety of natural language processing (NLP) and analysis tasks, including translating, classifying, and generating text; answering questions in a conversational manner; and identifying data patterns.
- For example, virtual assistants like Siri, Alexa, or Google Assistant use LLMs to process natural language queries and provide useful information or execute tasks such as setting reminders or controlling smart home devices.
- Agentic AI:
- Agentic AI is an artificial intelligence system that can accomplish a specific goal with limited supervision. It consists of AI agents—machine learning models that mimic human decision-making to solve problems in real time. In a multiagent system, each agent performs a specific subtask required to reach the goal and their efforts are coordinated through AI orchestration.
- Unlike traditional AI models, which operate within predefined constraints and require human intervention, agentic AI exhibits autonomy, goal-driven behavior and adaptability. The term “agentic” refers to these models’ agency, or, their capacity to act independently and purposefully.
- Agentic AI builds on generative AI (gen AI) techniques by using large language models (LLMs) to function in dynamic environments. While generative models focus on creating content based on learned patterns, agentic AI extends this capability by applying generative outputs toward specific goals.
- EBITA:
- Earnings before interest, taxes, and amortization (EBITA) is a measure of company profitability used by investors. It is helpful for comparing one company to another in the same line of business.
- EBITA = Net income + Interest + Taxes + Amortization
- EBITDA:
- Earnings before interest, taxes, depreciation, and amortization (EBITDA) is an alternate measure of profitability to net income. By including depreciation and amortization as well as taxes and debt payment costs, EBITDA attempts to represent the cash profit generated by the company’s operations.
- EBITDA and EBITA are both measures of profitability. The difference is that EBITDA also excludes depreciation.
- EBITDA is the more commonly used measure because it adds depreciation—the accounting practice of recording the reduced value of a company’s tangible assets over time—to the list of factors.
- EV/EBITDA (Enterprise Multiple):
- Enterprise multiple, also known as the EV-to-EBITDA multiple, is a ratio used to determine the value of a company.
- It is computed by dividing enterprise value by EBITDA.
- The enterprise multiple takes into account a company’s debt and cash levels in addition to its stock price and relates that value to the firm’s cash profitability.
- Enterprise multiples can vary depending on the industry.
- Higher enterprise multiples are expected in high-growth industries and lower multiples in industries with slow growth.
- Free Cash Flow (FCF):
- Free cash flow (FCF) is a company’s available cash repaid to creditors and as dividends and interest to investors. Management and investors use free cash flow as a measure of a company’s financial health. FCF reconciles net income by adjusting for non-cash expenses, changes in working capital, and capital expenditures. Free cash flow can reveal problems in the financial fundamentals before they become apparent on a company’s income statement. A positive free cash flow doesn’t always indicate a strong stock trend. FCF is money that is on hand and free to use to settle liabilities or obligations.
- PLC (Programmable Logic Controller):
- Programmable Logic Controllers (PLCs) are industrial computers, with various inputs and outputs, used to control and monitor industrial equipment based on custom programming.
- Receive information from input devices or sensors, process the data, and perform specific tasks or output specific information based on pre-programmed parameters. PLCs are often used to do things like monitor machine productivity, track operating temperatures, and automatically stop or start processes. They are also often used to trigger alarms if a machine malfunctions.
- A PLC takes in inputs, whether from automated data capture points or from human input points such as switches or buttons. Based on its programming, the PLC then decides whether or not to change the output. A PLC’s outputs can control a huge variety of equipment, including motors, solenoid valves, lights, switchgear, safety shut-offs and many others.
- PLC can be used for motion control, but with difficult programming and complexity; whereas a motion controller can be used for process automation too with equal easiness.
- A PLC is a stand-alone unit that can control one or more machines and is connected to them by cables. On the other hand, in an embedded control architecture the controller — which is almost always a printed circuit board (PCB) — is located inside the machine it controls.
- Embedded PCs – Industrial PC (IPC):
- Embedded computer systems go by many names (Box PC, Gateway, Controller, Industrial PC, etc), but an Embedded PC is essentially any specialized computer system that is implemented as part of a larger device, intelligent system, or installation.
- Embedded Devices are less complex devices than Computers. Computers may be installed in other devices but are self-sufficient to exist. Embedded Devices only exist inside other Systems. Computers are more Difficult when used, compared to an Embedded System.