Danfoss – Danfoss completes acquisition of ENFOR’s district energy efficiency software
Danfoss A/S has acquired ENFOR’s district energy software business, with plans to scale its advanced AI driven energy efficiency optimization worldwide, to enable the decarbonization of cities.
Danfoss has acquired ENFOR’s district energy software and will bring the solutions to the global market under the Danfoss Leanheat® suite of sustainable heating and cooling solutions. The Danfoss Leanheat® solution combines cutting-edge technology, data analytics, and artificial intelligence to optimize energy consumption and improve operational efficiency of district energy and buildings. Danfoss had been a minority shareholder in ENFOR since 2020. By fully acquiring ENFOR’s district heating software business, Danfoss enhances the capabilities and accuracy of its Leanheat network suite for district energy utilities to include data-driven temperature optimization, intelligent load forecasting and micro weather forecasting, which support district energy utilities and energy companies with their green transitions.
ENFOR is an innovative spin-off from the Danish Technical University and delivers solutions for forecasting and optimization of energy production and demand, incl. optimization of district energy systems. The potential of using data and machine learning in the energy sector is massive. The latest Danfoss Impact White Paper reveals that an ambitious but realistic roll out of demand-side flexibility technology in the EU and UK can save 40 million tons of CO2 emissions each year by 2030, more than Denmark’s domestic climate footprint.
With countless solutions on the market focused on siloed changes, utilities, building owners, and public stakeholders are left without a holistic solution. Danfoss Leanheat® offers innovative end-to-end software systems and services for the control and optimization of district energy systems—from people to production. ENFOR’s solution will be a core part of Danfoss Leanheat® and going forward the Danfoss team will continuously evolve the combined solution to develop new features to meet the needs of the evolving market.
After the separation of the district heating activities, ENFOR will continue as an independent legal entity and will focus on developing its advanced machine learning technologies for the electricity sector, with a dedication to renewables and the green energy transition.
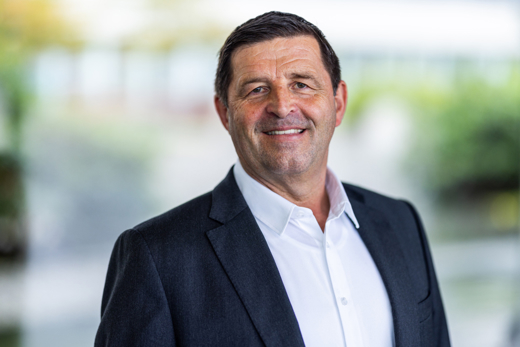
Jürgen Fischer, President Danfoss Climate Solutions, says: “We are thrilled to welcome ENFOR’s district energy experts and software to Danfoss. Together we can offer a complete solution to utilities for measuring and optimizing the energy efficiency of district energy networks, to better serve our customers. For Danfoss, scaling and enhancing our digital portfolio and offerings is a key priority. By unlocking the potential of artificial intelligence, connectivity, and optimization tools, we make it possible for the utilities and service providers to effectively meet the growing demands for energy efficiency while improving business operations and costs.”
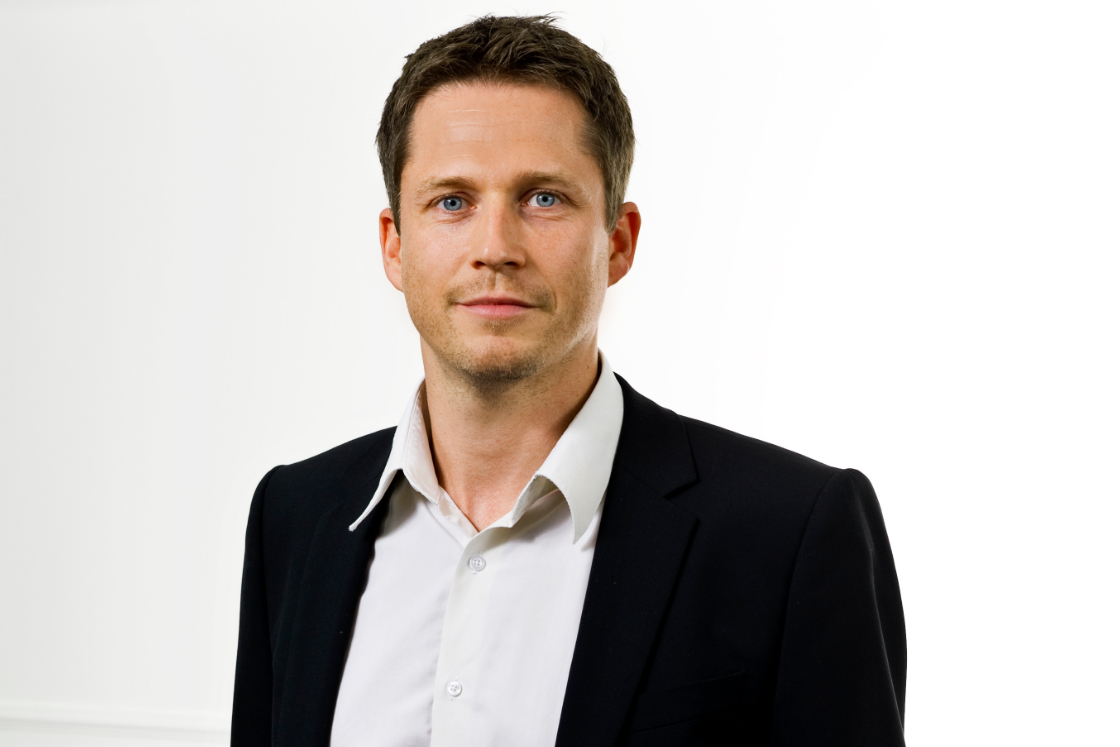
Mikkel Westenholz, CEO ENFOR A/S, says: “We are happy that our district heating services have found a new home with Danfoss, who will be able to integrate the services into their digital platform, and bring the services to the market through their global organization. It has been an exciting journey for ENFOR, where we were an early pioneer for the use of data and machine learning in forecasting and optimization of energy systems. We see it as a strong validation of our business and technology, that a company like Danfoss has acquired our district heating and cooling technology, and we will continue our journey with a focus on renewable energy and the power sector.”
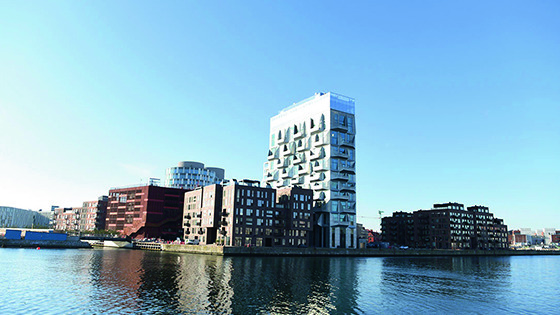
SourceDanfoss
EMR Analysis
More information on Danfoss: See the full profile on EMR Executive Services
More information on Kim Fausing (President and Chief Executive Officer, Danfoss): See the full profile on EMR Executive Services
More information on Jürgen Fischer (Member of the Group Executive Team (GET), President, Danfoss Climate Solutions, Danfoss): See the full profile on EMR Executive Services
More information on Leanheat® by Danfoss: https://leanheat.com/ + Leanheat’s development began in 2011. The first sites with Internet of Things (IoT) and artificial intelligence (AI) technologies were taken into use in 2013. And officially Leanheat as a company was founded in 2016. Since then, growth has been vigorous. Leanheat is now installed in around 180,000 apartments. It is the world’s largest IoT solution for collective heating.
Fully automated and self-learning, Leanheat’s solution provides real-time optimization not only for individual buildings, but entire clusters of apartment buildings – while at the same time giving the opportunity for district heating companies to serve their customers more efficiently and with a smaller carbon footprint.
We work in collaboration with industry pioneers such as Fortum and Danfoss. Piloted in Finland with its harsh climate conditions, we are now looking at rapid international expansion.
More information on ENFOR A/S: https://enfor.dk + ENFOR provides market-leading energy forecasting and optimization solutions for the energy sector. Utilities, energy traders, transmission and distribution system operators use ENFOR solutions for forecasting of renewable energy production, electricity and heat demand as well as optimization of district heating systems. ENFOR was established in 2006 as a spin-off from the Technical University of Denmark. The company has a solid operational track record and has successfully served customers all over the world for many years.
More information on Mikkel Westenholz (Chief Executive Officer, ENFOR): https://enfor.dk/company/about-enfor/ + https://www.linkedin.com/in/mikkel-westenholz/
EMR Additional Notes:
- AI – Artificial Intelligence:
- https://searchenterpriseai.techtarget.com/definition/AI-Artificial-Intelligence +
- Artificial intelligence is the simulation of human intelligence processes by machines, especially computer systems. Specific applications of AI include expert systems, natural language processing, speech recognition and machine vision.
- As the hype around AI has accelerated, vendors have been scrambling to promote how their products and services use AI. Often what they refer to as AI is simply one component of AI, such as machine learning. AI requires a foundation of specialized hardware and software for writing and training machine learning algorithms. No one programming language is synonymous with AI, but a few, including Python, R and Java, are popular.
- In general, AI systems work by ingesting large amounts of labeled training data, analyzing the data for correlations and patterns, and using these patterns to make predictions about future states. In this way, a chatbot that is fed examples of text chats can learn to produce lifelike exchanges with people, or an image recognition tool can learn to identify and describe objects in images by reviewing millions of examples.
- AI programming focuses on three cognitive skills: learning, reasoning and self-correction.
- What are the 4 types of artificial intelligence?
- Type 1: Reactive machines. These AI systems have no memory and are task specific. An example is Deep Blue, the IBM chess program that beat Garry Kasparov in the 1990s. Deep Blue can identify pieces on the chessboard and make predictions, but because it has no memory, it cannot use past experiences to inform future ones.
- Type 2: Limited memory. These AI systems have memory, so they can use past experiences to inform future decisions. Some of the decision-making functions in self-driving cars are designed this way.
- Type 3: Theory of mind. Theory of mind is a psychology term. When applied to AI, it means that the system would have the social intelligence to understand emotions. This type of AI will be able to infer human intentions and predict behavior, a necessary skill for AI systems to become integral members of human teams.
- Type 4: Self-awareness. In this category, AI systems have a sense of self, which gives them consciousness. Machines with self-awareness understand their own current state. This type of AI does not yet exist.
- Machine Learning:
- Developed to mimic human intelligence. It lets the machines learn independently by ingesting vast amounts of data and detecting patterns. Many ML algorithms use statistics formulas and big data to function.
- Type of artificial intelligence (AI) that allows software applications to become more accurate at predicting outcomes without being explicitly programmed to do so. Machine learning algorithms use historical data as input to predict new output values.
- Recommendation engines are a common use case for machine learning. Other popular uses include fraud detection, spam filtering, malware threat detection, business process automation (BPA) and Predictive maintenance.
- Classical machine learning is often categorized by how an algorithm learns to become more accurate in its predictions. There are four basic approaches: supervised learning, unsupervised learning, semi-supervised learning and reinforcement learning. The type of algorithm data scientists choose to use depends on what type of data they want to predict.
- Deep Learning:
- Subset of machine learning. Deep learning enabled much smarter results than were originally possible with machine learning. Consider the face recognition example.
- Deep learning makes use of layers of information processing, each gradually learning more and more complex representations of data. The early layers may learn about colors, the next ones learn about shapes, the following about combinations of those shapes, and finally actual objects. Deep learning demonstrated a breakthrough in object recognition.
- Deep learning is currently the most sophisticated AI architecture we have developed.
- Computer Vision:
- Computer vision is a field of artificial intelligence (AI) that enables computers and systems to derive meaningful information from digital images, videos and other visual inputs — and take actions or make recommendations based on that information.
- The most well-known case of this today is Google’s Translate, which can take an image of anything — from menus to signboards — and convert it into text that the program then translates into the user’s native language.
- Generative AI:
- Generative AI technology generates outputs based on some kind of input – often a prompt supplied by a person. Some GenAI tools work in one medium, such as turning text inputs into text outputs, for example. With the public release of ChatGPT in late November 2022, the world at large was introduced to an AI app capable of creating text that sounded more authentic and less artificial than any previous generation of computer-crafted text.
- https://searchenterpriseai.techtarget.com/definition/AI-Artificial-Intelligence +
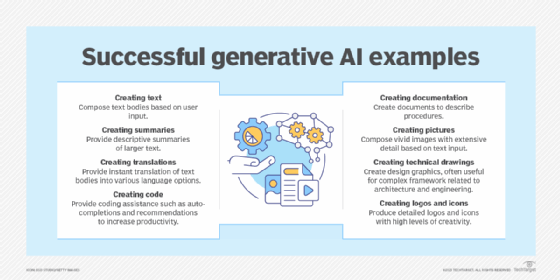
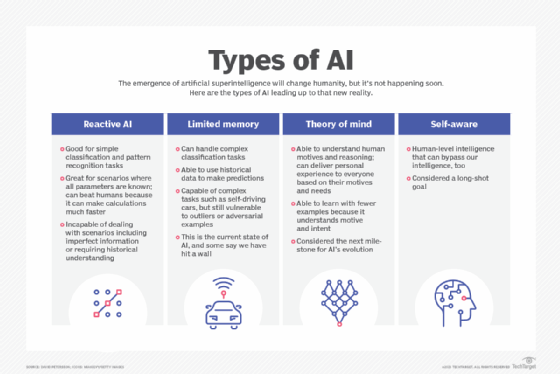
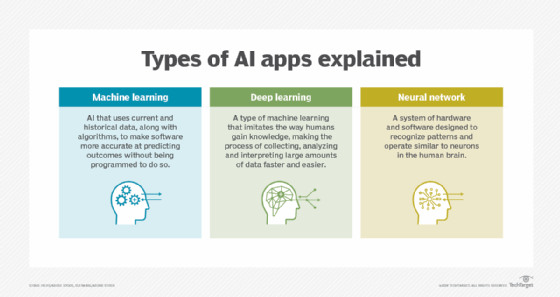
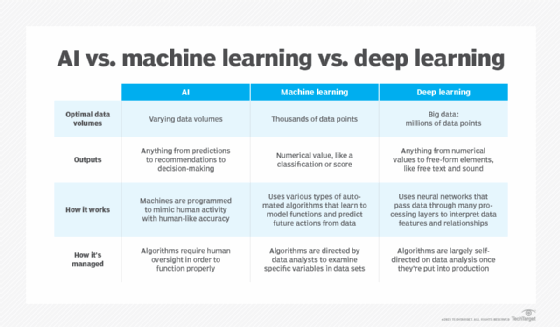
- Carbon Dioxide (CO2):
- Primary greenhouse gas emitted through human activities. Carbon dioxide enters the atmosphere through burning fossil fuels (coal, natural gas, and oil), solid waste, trees and other biological materials, and also as a result of certain chemical reactions (e.g., manufacture of cement). Carbon dioxide is removed from the atmosphere (or “sequestered”) when it is absorbed by plants as part of the biological carbon cycle.
- Biogenic Carbon Dioxide (CO2):
- Carbon Dioxide released as a result of the combustion or decomposition of organic material, that is biomass and its derivatives. Examples include carbon dioxide released during the combustion of wood and biogas generated by decomposition.
- Biogenic Carbon Dioxide (CO2) and Carbon Dioxide (CO2) are the same. Scientists differentiate between biogenic carbon (that which is absorbed, stored and emitted by organic matter like soil, trees, plants and grasses) and non-biogenic carbon (that found in all other sources, most notably in fossil fuels like oil, coal and gas).
- Carbon Capture and Storage (CCS):
- CCS involves the capture of carbon dioxide (CO2) emissions from industrial processes, such as steel and cement production, or from the burning of fossil fuels in power generation. This carbon is then transported from where it was produced, via ship or in a pipeline, and stored deep underground in geological formations.
- CCS projects typically target 90 percent efficiency, meaning that 90 percent of the carbon dioxide from the power plant will be captured and stored.
- Decarbonization:
- Reduction of carbon dioxide emissions through the use of low carbon power sources, achieving a lower output of greenhouse gasses into the atmosphere.
- Carbon Footprint:
- There is no universally agreed definition of what a carbon footprint is. A carbon footprint is generally understood to be the total amount of greenhouse gas (GHG) emissions that are directly or indirectly caused by an individual, organization, product, or service. These emissions are typically measured in tonnes of carbon dioxide equivalent (CO2e).
- In 2009, the Greenhouse Gas Protocol (GHG Protocol) published a standard for calculating and reporting corporate carbon footprints. This standard is widely accepted by businesses and other organizations around the world. The GHG Protocol defines a carbon footprint as “the total set of greenhouse gas emissions caused by an organization, directly and indirectly, through its own operations and the value chain.”